A Time-Space Efficiency Optimization Method for High-Resolution Remote Sensing Image Classification Algorithms
A remote sensing image and classification algorithm technology, applied in the field of computer image processing and remote sensing, can solve problems such as difficulty, insufficient memory, long processing time of remote sensing images, etc. Effect
- Summary
- Abstract
- Description
- Claims
- Application Information
AI Technical Summary
Problems solved by technology
Method used
Image
Examples
Embodiment 1
[0023] The invention discloses a method for optimizing the space-time efficiency of a high-resolution remote sensing image classification algorithm. The specific steps of the optimization algorithm are as follows:
[0024] A: The image data is divided into several pixel blocks, each pixel block is 512*512;
[0025] B: Use the RasterIO function in the GDAL function library to load the pixel set of the image block by block, and utilize the memory-mapped file technology to transfer the pixel data block by block to a new disk file;
[0026] C: Randomly select K pixels as the initial cluster center;
[0027] D: Use the memory-mapped file technology to read the pixel data of the first block from the disk file, and transmit it to the device side;
[0028] E: Calculate the distance between each pixel of the block and each cluster center on the device side, and classify according to the principle of similarity;
[0029] F: Send the classification results back to the host, and use mem...
Embodiment 2
[0036] Experiment purpose and method: In order to evaluate the feasibility and effectiveness of this application, this embodiment takes the K-Means algorithm as an example, using a cropped small image (600*600 pixels) and a whole scene image (6000*6000 pixels) were experimented, and for an image of 600*600 pixels, the time spent on a single iteration of the three strategies of "CPU+Memory", "CUDA+Memory" and "CUDA+MMF" were compared; for a 6000*6000 pixel SPOT-5 The entire scene image, the time spent on a single iteration of the three strategies of "CUDA+Memory", "CUDA+MMF" and "CUDA+Blocking".
[0037] Experimental results: as shown in Table 1:
[0038] Table 1 Execution time of four implementation strategies of K-Means clustering algorithm (unit: ms)
[0039]
[0040] It can be seen that the time spent by the "CUDA+Memory" and "CUDA+MMF" strategies is far less than the time spent by the "CPU+Memory" strategy, only about 3% of the latter, and the time spent by the "CUDA+M...
Embodiment 3
[0043] Experiment purpose and method: In order to evaluate the feasibility and effectiveness of this application, this embodiment takes the K-Means algorithm as an example, and performs "CUDA+ memory", "CUDA+ MMF" and "CUDA+blocking" three strategies to compare and evaluate the memory usage.
[0044] Experimental results:
[0045] As shown in Table 2, for a small image of 600*600 pixels, the memory occupied by the "CUDA+MMF" and "CUDA+Blocking" strategies is slightly smaller than the memory occupied by the "CUDA+Memory" strategy; for a medium-sized image of 3000*3000 pixels , the memory occupied by the "CUDA+MMF" strategy and the "CUDA+blocking" strategy is significantly smaller than the memory occupied by the "CUDA+Memory" strategy, only about 25% of the latter; for a large image of 6000*6000 pixels, "CUDA+MMF" And the memory occupied by the "CUDA+blocking" strategy is much smaller than the memory occupied by the "CUDA+memory" strategy, only about 10% of the latter. It can ...
PUM
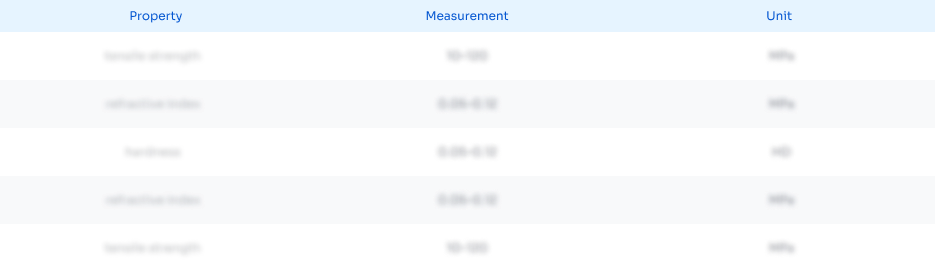
Abstract
Description
Claims
Application Information
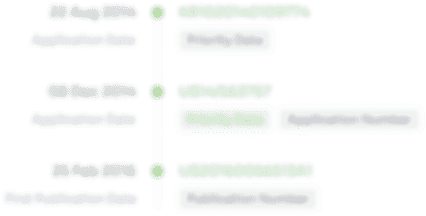
- Generate Ideas
- Intellectual Property
- Life Sciences
- Materials
- Tech Scout
- Unparalleled Data Quality
- Higher Quality Content
- 60% Fewer Hallucinations
Browse by: Latest US Patents, China's latest patents, Technical Efficacy Thesaurus, Application Domain, Technology Topic, Popular Technical Reports.
© 2025 PatSnap. All rights reserved.Legal|Privacy policy|Modern Slavery Act Transparency Statement|Sitemap|About US| Contact US: help@patsnap.com