A Method for Satisfaction Evaluation of Search Engine Users' Information Needs by Fusion of Multi-view and Semi-Supervised Learning
A semi-supervised learning and search engine technology, applied in the field of satisfaction evaluation of search engine user information needs that integrates multi-view and semi-supervised learning, can solve problems such as difficult real-time development, large consumption of manpower and time resources, ignoring nature, etc.
- Summary
- Abstract
- Description
- Claims
- Application Information
AI Technical Summary
Problems solved by technology
Method used
Image
Examples
Embodiment
[0067] Example: such as figure 1 As shown, the search engine user information demand satisfaction evaluation method that integrates multi-view and semi-supervised learning includes data preprocessing, training sub-view satisfaction model, assigning pseudo-labels to unlabeled data, and training based on multi-view and semi-supervised learning. Supervised Learning for User Satisfaction Modeling and Evaluation in Six Stages.
[0068] The data preprocessing stage includes two sub-stages: labeled data preprocessing and unlabeled data preprocessing:
[0069] The flow chart of the annotation data preprocessing stage is as follows: figure 2 As shown, it mainly includes the following steps:
[0070] Step 1. Divide search engine log data into behavior view data and time view data. Behavior view data describes the user's search process from the transition between user search behaviors, including three columns of data: information needs, search behavior, and satisfaction; time view da...
PUM
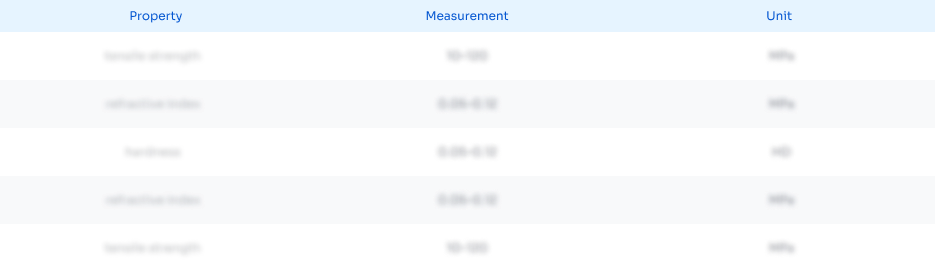
Abstract
Description
Claims
Application Information
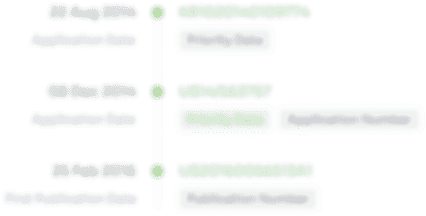
- R&D Engineer
- R&D Manager
- IP Professional
- Industry Leading Data Capabilities
- Powerful AI technology
- Patent DNA Extraction
Browse by: Latest US Patents, China's latest patents, Technical Efficacy Thesaurus, Application Domain, Technology Topic, Popular Technical Reports.
© 2024 PatSnap. All rights reserved.Legal|Privacy policy|Modern Slavery Act Transparency Statement|Sitemap|About US| Contact US: help@patsnap.com