Multitask learning object tracking method based on subspace characteristics
A multi-task learning and subspace feature technology, applied in the field of computer data and image processing, can solve the problems of target drift, large amount of calculation, and high dimensionality of over-complete dictionaries, and achieve overcoming illumination changes, reduced calculation amount, fast and robust tracked effect
- Summary
- Abstract
- Description
- Claims
- Application Information
AI Technical Summary
Problems solved by technology
Method used
Image
Examples
Embodiment Construction
[0025] Now in conjunction with embodiment, accompanying drawing, the present invention will be further described:
[0026] On the basis of determining the target in the first frame, the present invention first uses the PCA subspace feature representation method to represent the target, and introduces trivial templates to construct a dictionary for sparse representation. Secondly, the candidate targets generated by the particle filter method are jointly sparsely represented, and the representation coefficients are obtained by solving. Finally, the candidate target with the smallest reconstruction error is selected as the tracking result. The specific steps are as follows, and the process can refer to the accompanying drawings.
[0027] 1) Read the first frame of image data and the parameters [x, y, w, h] of the target block in the first frame of the image, randomly select m particle points around the target according to the Gaussian distribution (m takes 600), and record Its ...
PUM
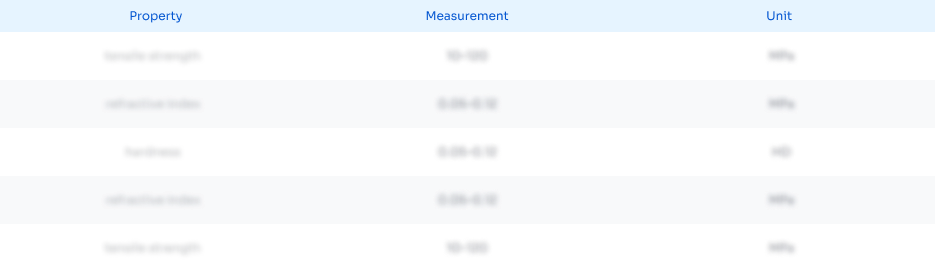
Abstract
Description
Claims
Application Information
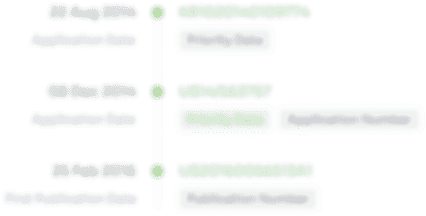
- R&D Engineer
- R&D Manager
- IP Professional
- Industry Leading Data Capabilities
- Powerful AI technology
- Patent DNA Extraction
Browse by: Latest US Patents, China's latest patents, Technical Efficacy Thesaurus, Application Domain, Technology Topic, Popular Technical Reports.
© 2024 PatSnap. All rights reserved.Legal|Privacy policy|Modern Slavery Act Transparency Statement|Sitemap|About US| Contact US: help@patsnap.com