Hyperspectral remote sensing data feature extraction method based on convolution neural network
A convolutional neural network and hyperspectral remote sensing technology, applied in the field of remote sensing data feature extraction, can solve problems such as unfavorable image processing and feature data without deep structure
- Summary
- Abstract
- Description
- Claims
- Application Information
AI Technical Summary
Problems solved by technology
Method used
Image
Examples
specific Embodiment approach 1
[0015] Specific implementation mode one: the following combination figure 1 Illustrate this embodiment, the hyperspectral remote sensing data feature extraction method based on convolutional neural network described in this embodiment, it comprises the following steps:
[0016] Step 1: Perform bad band removal, data deformation reorganization and data preprocessing on the hyperspectral raw data to obtain standardized input data;
[0017] Step 2: The standardized input data is used as the input of the convolutional neural network convolution layer, and the standardized input data is convoluted through n trainable filters and offsets to obtain n different feature maps, where n is Positive integer; each neuron in each feature map is connected to a local receptive field of standardized input data for extracting corresponding local features, and the corresponding local features obtained by all neurons in each feature map are integrated Finally, a global information is obtained, an...
specific Embodiment approach 2
[0025] Embodiment 2: This embodiment further explains Embodiment 1. The removal of bad bands specifically includes: deleting bands in the original hyperspectral data whose bands are all zero values or whose noise exceeds a preset noise threshold, and obtain a three-dimensional matrix. No bad bands to enter data.
[0026] In the process of hyperspectral imaging, there are some bands that are all zero or noisy due to water absorption or other reasons. In the bad band removal part, these bands need to be deleted to avoid singularity in subsequent data processing and meet the needs of subsequent algorithms.
specific Embodiment approach 3
[0027] Specific implementation mode three: this implementation mode further explains implementation mode two, and the data deformation and reorganization is specifically: extracting the pixel of each pixel point from the input data without bad bands in order from left to right and from top to bottom Vector, each pixel vector is used as a row vector, and all the row vectors are arranged downwards in order to form a two-dimensional matrix input data. The number of rows of the two-dimensional matrix input data is the number of pixels of the input data without bad bands. The number of columns is the number of bands each pixel contains.
[0028] In this embodiment, the original hyperspectral data after bad band removal is reorganized to meet the data input requirements of the convolutional neural network. The original hyperspectral data can be regarded as a three-dimensional matrix, and the vector formed by different band positions at the same spatial position is called a pixel vec...
PUM
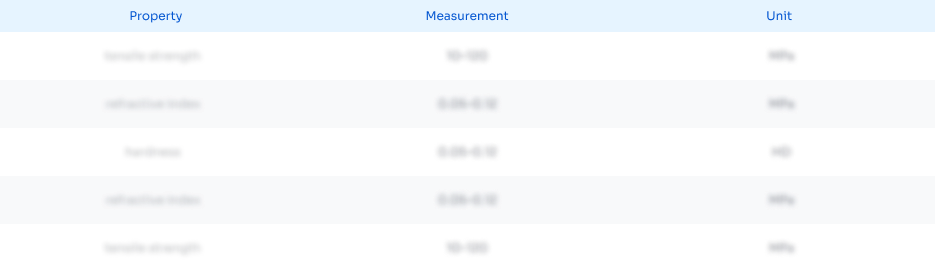
Abstract
Description
Claims
Application Information
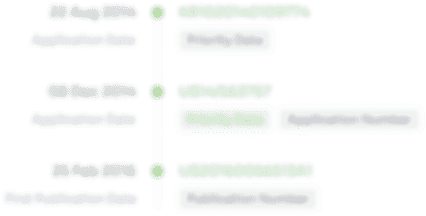
- R&D Engineer
- R&D Manager
- IP Professional
- Industry Leading Data Capabilities
- Powerful AI technology
- Patent DNA Extraction
Browse by: Latest US Patents, China's latest patents, Technical Efficacy Thesaurus, Application Domain, Technology Topic, Popular Technical Reports.
© 2024 PatSnap. All rights reserved.Legal|Privacy policy|Modern Slavery Act Transparency Statement|Sitemap|About US| Contact US: help@patsnap.com