Hyperspectral Image Compressive Sensing Method Based on Reweighted Laplacian Sparse Prior
A hyperspectral image and sparse prior technology, which is applied in the field of hyperspectral image compression sensing based on reweighted Laplacian sparse prior, can solve the problem of low reconstruction accuracy
- Summary
- Abstract
- Description
- Claims
- Application Information
AI Technical Summary
Problems solved by technology
Method used
Image
Examples
Embodiment Construction
[0060] The specific steps of the hyperspectral image compressed sensing method based on the heavy-weighted Laplacian sparse prior of the present invention are as follows:
[0061] Will contain n b Bands, each band contains n p Each band of the hyperspectral image of pixels is stretched into a row vector, and all row vectors form a two-dimensional matrix. Among them, each column of X represents the spectrum corresponding to each pixel, and this direction is the spectral dimension; each row of X corresponds to all the pixel values of a band, and this direction is the spatial dimension. The present invention mainly includes the following four steps, which are specifically as follows:
[0062] 1. Obtain compressed data.
[0063] Gaussian random observation matrix with column normalization Sampling the spectral dimension of hyperspectral image X to obtain compressed data m b Indicates the length of the band after compression, ρ=m b / n b Is the sampling rate.
[0064] G=AX+N (1) where,...
PUM
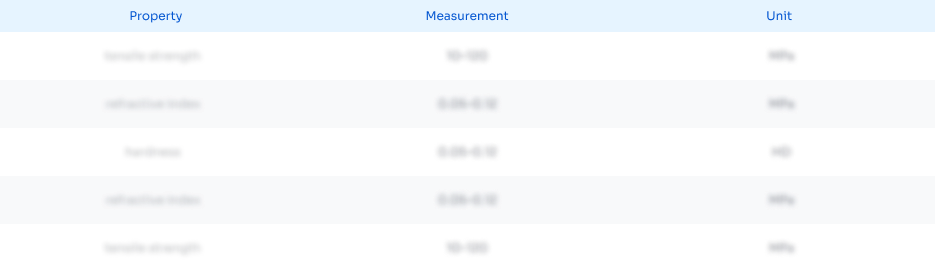
Abstract
Description
Claims
Application Information
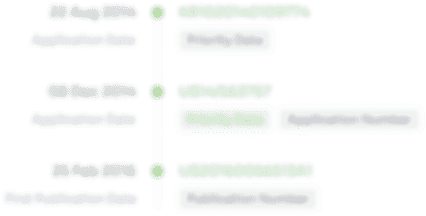
- R&D Engineer
- R&D Manager
- IP Professional
- Industry Leading Data Capabilities
- Powerful AI technology
- Patent DNA Extraction
Browse by: Latest US Patents, China's latest patents, Technical Efficacy Thesaurus, Application Domain, Technology Topic.
© 2024 PatSnap. All rights reserved.Legal|Privacy policy|Modern Slavery Act Transparency Statement|Sitemap