Brain electrical signal independent component extraction method based on convolution blind source separation
An EEG signal and independent component technology, applied in instruments, character and pattern recognition, computer components, etc., can solve problems that affect the accuracy of EEG feature extraction and classification recognition, and cannot truly describe the mixing process of EEG signals. , to achieve the effect of improving accuracy, simple implementation, and good separation effect
- Summary
- Abstract
- Description
- Claims
- Application Information
AI Technical Summary
Problems solved by technology
Method used
Examples
Embodiment Construction
[0033] A method for extracting independent components of EEG signals based on convolutional blind source separation, the specific steps of which are:
[0034] The first step is to build an independent component extraction system for EEG signals based on convolutional blind source separation
[0035]EEG signal independent component extraction system based on convolutional blind source separation, including: AD sampling module, short-time Fourier transform module, frequency-domain instantaneous blind source separation module, sequence adjustment module and short-time Fourier transform module. The AD sampling module is used to sample the EEG signal to discretize the EEG signal; the short-time Fourier transform module is used to convert the convolution mixed signal in the time domain into an instantaneous mixed signal in the frequency domain; The blind source separation module is used to perform blind source separation on the instantaneous mixed signal in the frequency domain; the...
PUM
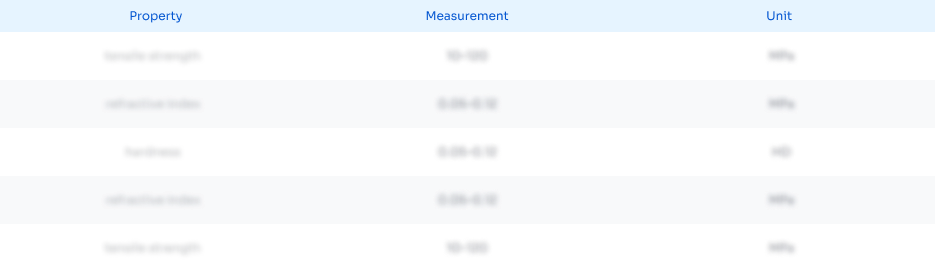
Abstract
Description
Claims
Application Information
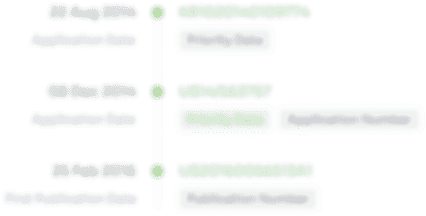
- R&D Engineer
- R&D Manager
- IP Professional
- Industry Leading Data Capabilities
- Powerful AI technology
- Patent DNA Extraction
Browse by: Latest US Patents, China's latest patents, Technical Efficacy Thesaurus, Application Domain, Technology Topic, Popular Technical Reports.
© 2024 PatSnap. All rights reserved.Legal|Privacy policy|Modern Slavery Act Transparency Statement|Sitemap|About US| Contact US: help@patsnap.com