Sequence deeply convinced network-based pedestrian identifying method
A technology of deep belief network and pedestrian recognition, which is applied in the field of pedestrian recognition based on sequence deep belief network, and can solve problems such as redundant information, lengthy generation process, discontinuity, etc.
- Summary
- Abstract
- Description
- Claims
- Application Information
AI Technical Summary
Problems solved by technology
Method used
Image
Examples
Embodiment Construction
[0049] In this example, if figure 1 As shown, the pedestrian recognition method based on the sequence deep belief network applied to the sequence deep belief network including the pedestrian database includes the following steps: preprocessing the training images in the pedestrian database, obtaining the required training sample images, and performing training on the obtained training images Extract the HOG feature of the sample image, construct and train the sequence deep belief network based on the sequence restricted Boltzmann machine, and use the sequence deep belief network to further extract the obtained HOG features to form the feature vector of the training sample, and the obtained The feature data is input into the support vector machine classifier to complete the training; the pedestrian image to be tested is preprocessed to obtain the test sample, and the pedestrian feature extraction is performed on the test sample with the HOG used in the training process and the s...
PUM
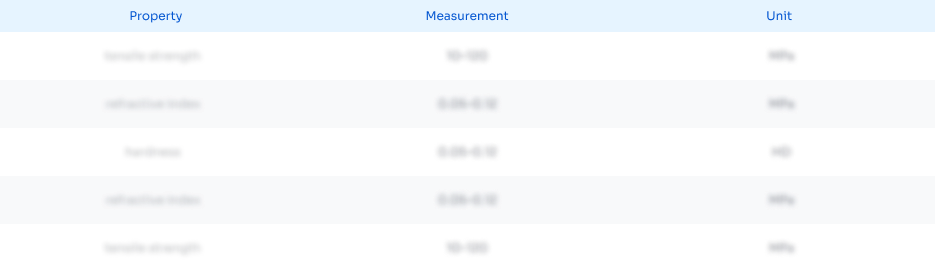
Abstract
Description
Claims
Application Information
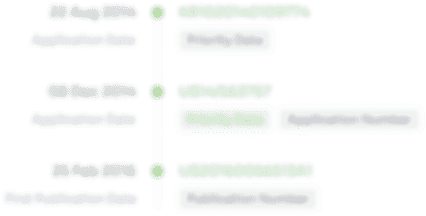
- Generate Ideas
- Intellectual Property
- Life Sciences
- Materials
- Tech Scout
- Unparalleled Data Quality
- Higher Quality Content
- 60% Fewer Hallucinations
Browse by: Latest US Patents, China's latest patents, Technical Efficacy Thesaurus, Application Domain, Technology Topic, Popular Technical Reports.
© 2025 PatSnap. All rights reserved.Legal|Privacy policy|Modern Slavery Act Transparency Statement|Sitemap|About US| Contact US: help@patsnap.com