A Short-Term Wind Speed Forecasting Method Based on Deep Neural Network Migration Model
A technology of deep neural network and wind speed, applied in the direction of biological neural network model, neural learning method, etc., can solve the problem of less data in new wind farms, and achieve the effect of improving prediction accuracy and strong feature learning ability
- Summary
- Abstract
- Description
- Claims
- Application Information
AI Technical Summary
Problems solved by technology
Method used
Image
Examples
Embodiment Construction
[0031] The technical solutions of the present invention will be further described in detail below in conjunction with the accompanying drawings and specific embodiments.
[0032] The invention proposes a model for short-term wind speed forecasting based on a deep neural network migration model. Using deep learning technology to build a multi-input multi-output deep neural network model with a shared hidden layer, such as figure 1 shown. In this structure, the input layer and hidden layer are shared by all wind farms, which can be regarded as a common feature transformation. The output layer is independent of each wind farm because their data distributions are different. This is a type of knowledge transfer, as common features are transferred to each dataset.
[0033] Such as figure 1 As shown, the present invention proposes a short-term wind speed forecasting method based on deep neural network transfer model, it is characterized in that, comprises the following steps:
...
PUM
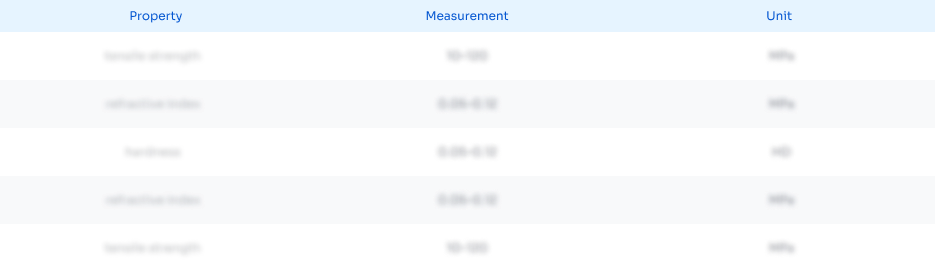
Abstract
Description
Claims
Application Information
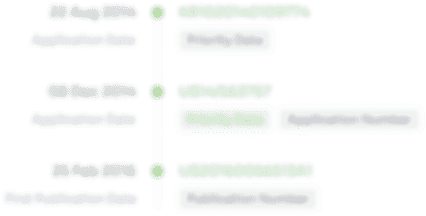
- R&D Engineer
- R&D Manager
- IP Professional
- Industry Leading Data Capabilities
- Powerful AI technology
- Patent DNA Extraction
Browse by: Latest US Patents, China's latest patents, Technical Efficacy Thesaurus, Application Domain, Technology Topic, Popular Technical Reports.
© 2024 PatSnap. All rights reserved.Legal|Privacy policy|Modern Slavery Act Transparency Statement|Sitemap|About US| Contact US: help@patsnap.com