Extreme learning machine-based pipeline magnetic flux leakage defect detection method
An extreme learning machine and magnetic flux leakage technology, applied in the direction of material magnetic variables, etc., to achieve the effect of predicting pipeline risks, fast learning speed, and good generalization performance
- Summary
- Abstract
- Description
- Claims
- Application Information
AI Technical Summary
Problems solved by technology
Method used
Image
Examples
Embodiment Construction
[0022] The specific embodiments of the present invention will be described in detail below in conjunction with the accompanying drawings.
[0023] A pipeline magnetic flux leakage defect detection method based on extreme learning machine, such as figure 1 shown, including the following steps:
[0024] Step 1: Obtain the shape parameters of the known pipeline magnetic flux leakage defect, including the length, width and depth data of the pipeline magnetic flux leakage defect, and extract the eigenvalues of the magnetic flux leakage signal waveform at the known pipeline magnetic flux leakage defect to extract the magnetic flux leakage Signal waveform eigenvalues.
[0025] Step 1.1: Obtain the shape parameters of the known pipeline magnetic flux leakage defect, including the length, width and depth data of the pipeline magnetic flux leakage defect, obtain the defect signal waveform diagram of the known pipeline magnetic flux leakage defect, and obtain the horizontal and vertic...
PUM
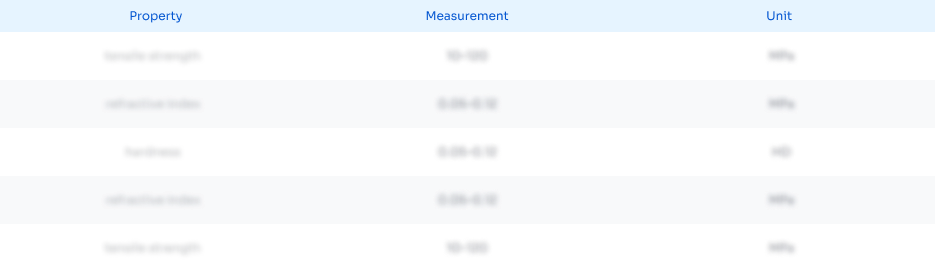
Abstract
Description
Claims
Application Information
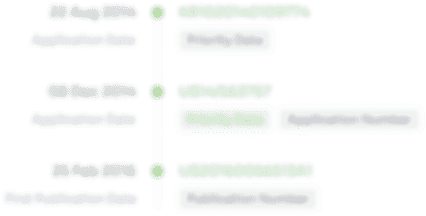
- R&D Engineer
- R&D Manager
- IP Professional
- Industry Leading Data Capabilities
- Powerful AI technology
- Patent DNA Extraction
Browse by: Latest US Patents, China's latest patents, Technical Efficacy Thesaurus, Application Domain, Technology Topic, Popular Technical Reports.
© 2024 PatSnap. All rights reserved.Legal|Privacy policy|Modern Slavery Act Transparency Statement|Sitemap|About US| Contact US: help@patsnap.com