Integrated classifier and classification method thereof
A technology that integrates classifiers and classification methods, which is applied in the direction of machine executive devices, instruments, character and pattern recognition, etc., can solve the problems of slow speed and low precision, and achieve the effect of maintaining the integrity of decision-making
- Summary
- Abstract
- Description
- Claims
- Application Information
AI Technical Summary
Problems solved by technology
Method used
Image
Examples
specific Embodiment approach 1
[0045] Specific implementation mode 1. Combination figure 1 and figure 2 Specifically illustrate this embodiment, the classification method of the integrated classifier described in this embodiment, it comprises the following steps:
[0046] Step 1. Read the raster data to be processed by means of a combination of multi-process and multi-thread. The specific process includes the following steps:
[0047] A. Input the number n of sub-classifiers of the integrated classifier;
[0048] n is the number of sub-classifiers, n is greater than or equal to 2, all the spatial attributes of the raster data are divided into n parts according to the decision-making ability through the expectation algorithm, each classifier has all the classification capabilities of the complete set,
[0049] B. Start n+1 processes;
[0050] Among them, the n+1 processes are Rank 0, Rank 1...Rankn; Rank0 is the management process, and Rank 1...Rankn is the operation process, and the operation process Ra...
specific Embodiment approach 2
[0059] Embodiment 2. The difference between this embodiment and the classification method with integrated classifiers described in Embodiment 1 is that the raster data described in step A is high-dimensional raster data.
[0060] In this embodiment, for massive high-dimensional raster data, traditional algorithms are slow in speed and low in precision. However, this patent achieves fast processing of raster data and acquisition of classification models. Moreover, due to the adoption of a heterogeneous decision-making mechanism, the classification accuracy is also high.
specific Embodiment approach 3
[0061] Specific embodiment three, combine image 3 This embodiment is specifically described. The difference between this embodiment and the classification method of the integrated classifier described in Embodiment 1 or Embodiment 2 is that each thread described in step 2 starts to discretize the raster data of the corresponding spatial continuous attribute The specific steps are:
[0062] Step 21. Set the number of clusters to ceil;
[0063] Step 22. Find the initial center of uniformly distributed clustering between the maximum value and the minimum value of the spatial continuity attribute started by the thread;
[0064] Step two and three, according to the K-Means algorithm, cluster the initial center of the evenly distributed cluster to form ceil clusters;
[0065] Step 24, output its minimum and maximum values for each cluster to form ceil range intervals;
[0066] Step 25: Construct the ceil value range intervals into an interval list.
[0067] In this embodiment...
PUM
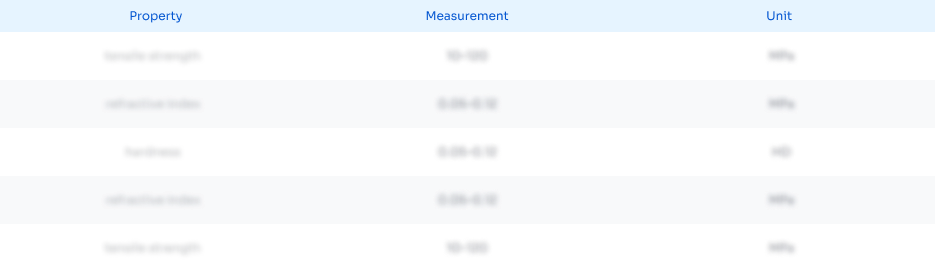
Abstract
Description
Claims
Application Information
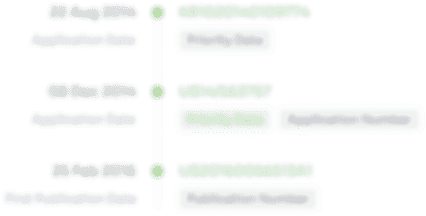
- R&D Engineer
- R&D Manager
- IP Professional
- Industry Leading Data Capabilities
- Powerful AI technology
- Patent DNA Extraction
Browse by: Latest US Patents, China's latest patents, Technical Efficacy Thesaurus, Application Domain, Technology Topic, Popular Technical Reports.
© 2024 PatSnap. All rights reserved.Legal|Privacy policy|Modern Slavery Act Transparency Statement|Sitemap|About US| Contact US: help@patsnap.com