Fuzzy clustering image segmenting method with transfer learning function
A technology of transfer learning and fuzzy clustering, applied in image analysis, image data processing, character and pattern recognition, etc., can solve problems such as low image segmentation accuracy, unsatisfactory segmentation accuracy, and image information loss
- Summary
- Abstract
- Description
- Claims
- Application Information
AI Technical Summary
Problems solved by technology
Method used
Image
Examples
Embodiment 1
[0076] Figure 4 The effect diagram is that by using such as figure 2 (b) The composite image with two texture features that is slightly polluted by noise is obtained at the iteration threshold ε=1e-7, the blur index m=1.1, the maximum number of iterations L=500, and the historical knowledge usage degree λ=0.01 It is only the preferred embodiment, but the present invention should not be limited to the content disclosed in the embodiment and the accompanying drawings. Therefore, all equivalents or modifications accomplished without departing from the disclosed spirit of the present invention fall into the protection scope of the present invention.
Embodiment 2
[0078] Figure 5 The effect diagram is that by using such as figure 2 (c) The composite image with two texture features that is moderately polluted by noise is obtained at the iteration threshold ε=1e-7, the blur index m=1.1, the maximum number of iterations L=500, and the historical knowledge usage degree λ=0.05 It is only the preferred embodiment, but the present invention should not be limited to the content disclosed in the embodiment and the accompanying drawings. Therefore, all equivalents or modifications accomplished without departing from the disclosed spirit of the present invention fall into the protection scope of the present invention.
PUM
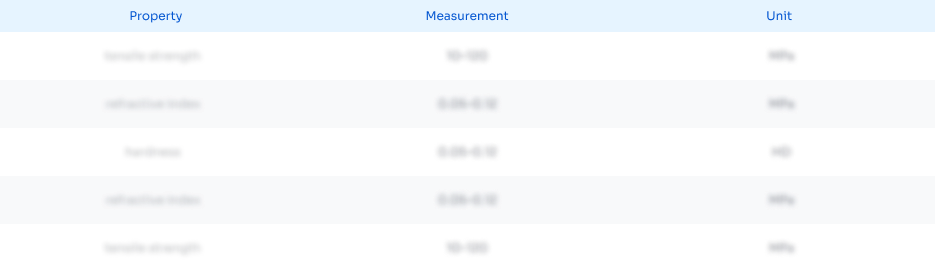
Abstract
Description
Claims
Application Information
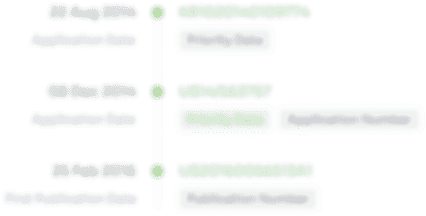
- R&D Engineer
- R&D Manager
- IP Professional
- Industry Leading Data Capabilities
- Powerful AI technology
- Patent DNA Extraction
Browse by: Latest US Patents, China's latest patents, Technical Efficacy Thesaurus, Application Domain, Technology Topic, Popular Technical Reports.
© 2024 PatSnap. All rights reserved.Legal|Privacy policy|Modern Slavery Act Transparency Statement|Sitemap|About US| Contact US: help@patsnap.com