Wind Power Forecasting Method Based on Continuous Time Period Clustering and Support Vector Machine Modeling
A technology of wind power prediction and support vector machine, which is applied to computer components, character and pattern recognition, instruments, etc., can solve the lack of classification of modeling data, the inability to meet wind power grid connection, and the similarity and prediction of training samples in the prediction model The accuracy is not ideal and other problems, to achieve the effect of strong practicality and promotion, improve similarity, and improve applicability
- Summary
- Abstract
- Description
- Claims
- Application Information
AI Technical Summary
Problems solved by technology
Method used
Image
Examples
Embodiment Construction
[0041] A wind power prediction method based on continuous time period clustering and support vector machine modeling, including the following steps:
[0042] ① Carry out unsupervised clustering of similar days throughout the year according to wind characteristics, including the following specific steps:
[0043] 1. According to the change trend, amplitude and volatility of wind speed in a day, construct classification samples. The sample structure form is as follows:
[0044]
[0045] In the formula, a s1 …a sH is the wind speed value at each time point in a day; a smax is the daily maximum wind speed; a smin is the daily minimum wind speed; a smean is the daily average wind speed; a sstd is the daily wind speed standard deviation;
[0046] 2. After determining the sample composition, each physical quantity needs to be normalized separately to eliminate the influence of different physical quantities on the clustering results due to dimensional differences. The n...
PUM
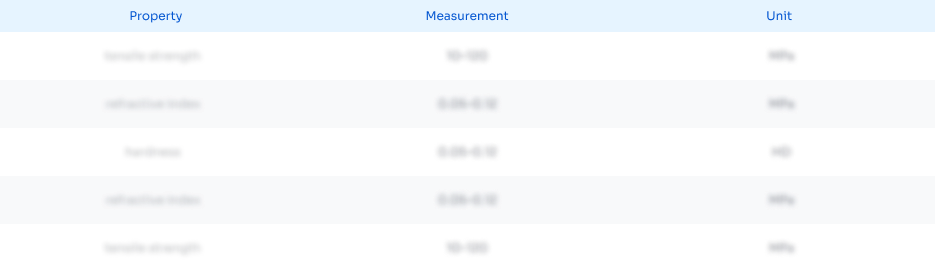
Abstract
Description
Claims
Application Information
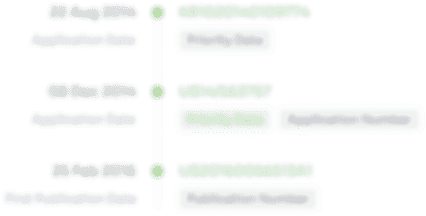
- R&D
- Intellectual Property
- Life Sciences
- Materials
- Tech Scout
- Unparalleled Data Quality
- Higher Quality Content
- 60% Fewer Hallucinations
Browse by: Latest US Patents, China's latest patents, Technical Efficacy Thesaurus, Application Domain, Technology Topic, Popular Technical Reports.
© 2025 PatSnap. All rights reserved.Legal|Privacy policy|Modern Slavery Act Transparency Statement|Sitemap|About US| Contact US: help@patsnap.com