Autonomous equipment decision control method based on distributed reinforcement learning
A technology of autonomous equipment and reinforcement learning, applied in the direction of adaptive control, comprehensive factory control, general control system, etc., can solve problems such as difficult to achieve results, limited ability of deep reinforcement learning model, and achieve the effect of avoiding slow training speed
- Summary
- Abstract
- Description
- Claims
- Application Information
AI Technical Summary
Problems solved by technology
Method used
Image
Examples
Embodiment Construction
[0043] Below in conjunction with specific embodiments, the present invention will be further illustrated, and it should be understood that these embodiments are only used to illustrate the present invention and not to limit the scope of the present invention. The modifications all fall within the scope defined by the appended claims of this application.
[0044] A decision-making control method for autonomous equipment based on distributed reinforcement learning. First, a training system including sampling nodes, cache nodes and training nodes is established, and then distributed asynchronous maximum entropy training is performed. Finally, the training results are compiled into efficient concurrent autonomous equipment decision-making. control module. Including training system building steps, distributed training steps and concurrent acceleration model export steps
[0045] The steps to build the training system are as follows: figure 1shown. In order to be able to run mult...
PUM
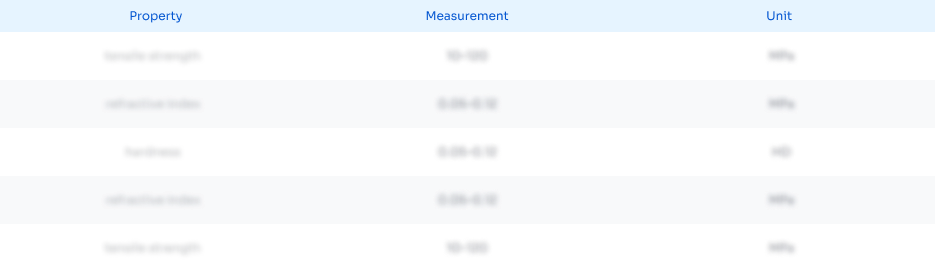
Abstract
Description
Claims
Application Information
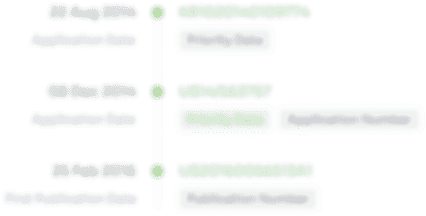
- R&D Engineer
- R&D Manager
- IP Professional
- Industry Leading Data Capabilities
- Powerful AI technology
- Patent DNA Extraction
Browse by: Latest US Patents, China's latest patents, Technical Efficacy Thesaurus, Application Domain, Technology Topic, Popular Technical Reports.
© 2024 PatSnap. All rights reserved.Legal|Privacy policy|Modern Slavery Act Transparency Statement|Sitemap|About US| Contact US: help@patsnap.com