Deep learning model deployment method and device, and medium
A deep learning and model technology, applied in the field of deep learning model deployment, can solve the problems of data sharing, difficult deployment, and difficult landing, and achieve the effects of fast inference, good stability, and good versatility
- Summary
- Abstract
- Description
- Claims
- Application Information
AI Technical Summary
Problems solved by technology
Method used
Image
Examples
Embodiment 1
[0055] Please refer to figure 1 and figure 2 , this embodiment mainly introduces a model training method on the Python side, by preprocessing the defect pictures obtained from the industrial camera and using the processed defect pictures to train the model;
[0056] Specifically, it includes the following steps:
[0057] S110. Defect image collection and defect labeling processing to generate a training data set.
[0058] S120. Expand the training data set; add defect types, and increase the training data set.
[0059] S130, dividing the training data set into training data and verification data;
[0060] Specifically, the training data set is randomly divided into training data and verification data in proportion;
[0061] Among them, the ratio of training data and validation data is 4:1.
[0062] S140. Select a model, train the model through the training data set, and adjust the training parameters according to the training effect;
[0063] Among them, the training pa...
Embodiment 2
[0072] Please refer to figure 1 and image 3 , this embodiment mainly introduces a Python-side model export method, including selecting a deployment framework type, exporting a model and a model description file; specifically, it includes the following steps:
[0073] S210. Select the deployment framework type;
[0074] S220, select a model format for the selected deployment framework type and then perform an export operation;
[0075] If you plan to deploy with the LibTorch framework, select PT for the model format in the export settings; if you plan to deploy with the TensorRT framework, select ONNX for the model format in the export settings;
[0076] S230, while exporting the model, generate a corresponding model description file;
[0077] The model description file contains: model task type (such as classification, positioning, segmentation or OCR), framework type (LibTorch, TensorRT), inference model type (accuracy priority, balance, speed priority), model loading ima...
Embodiment 3
[0080] Please refer to figure 1 , image 3 and Figure 4 , This embodiment mainly introduces a C++-side model part method, importing industrial pictures obtained from industrial cameras, automatically selecting the type of model deployment framework, and inferring and outputting detection results through model recognition: Specifically, it includes the following steps:
[0081] S310. Shooting through an industrial camera to obtain an industrial picture.
[0082] S320, load industrial pictures and model description files.
[0083] S330. Read the model description file, and automatically select corresponding inference parameters according to the model description file;
[0084] Specifically, read the model-related information (model task type, framework type, inference model type, model loading image length and width, and loading data arrangement format) in the model description file, and automatically set the corresponding inference parameters according to the model descript...
PUM
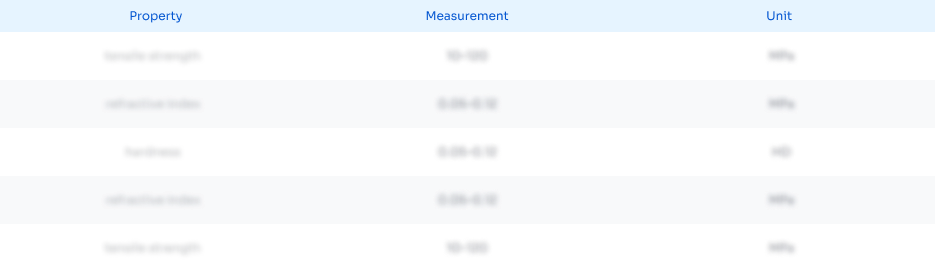
Abstract
Description
Claims
Application Information
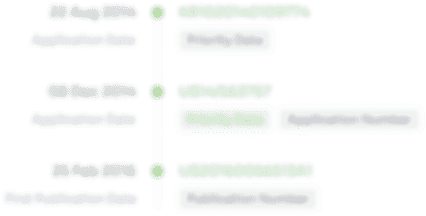
- R&D Engineer
- R&D Manager
- IP Professional
- Industry Leading Data Capabilities
- Powerful AI technology
- Patent DNA Extraction
Browse by: Latest US Patents, China's latest patents, Technical Efficacy Thesaurus, Application Domain, Technology Topic.
© 2024 PatSnap. All rights reserved.Legal|Privacy policy|Modern Slavery Act Transparency Statement|Sitemap