Multi-agent reinforcement learning method for collaborative decision-making of multiple combat units
A combat unit and multi-agent technology, applied in the direction of instruments, character and pattern recognition, computer components, etc., can solve the problems of low coordination of multi-combat unit decision-making and difficulty in obtaining valuable training samples, so as to increase diversity and Difficulty of battle, improvement of combat decision-making ability, and effect of synergistic effects
- Summary
- Abstract
- Description
- Claims
- Application Information
AI Technical Summary
Problems solved by technology
Method used
Image
Examples
Embodiment Construction
[0046] The present invention proposes a multi-agent enhanced learning method for multi-combat unit collaborative decision-making, such as figure 1 As shown, the steps include:
[0047] The first step is to establish a multi-agent reinforcement learning model for the red-blue game confrontation scenario, and realize intelligent collaborative decision-making modeling for multiple combat units.
[0048] The construction process of the multi-agent reinforcement learning model is as follows:
[0049] (1.1) Build a red and blue game confrontation scene;
[0050] (1.2) Analyze the task characteristics and decision points in the red and blue game confrontation scene, and determine the state space of the task decision point;
[0051] The specific method of state space design is as follows:
[0052] Before the modeling of the decision model, the overall situation information of the game confrontation scene and the local observation information of the combat unit are used as the stat...
PUM
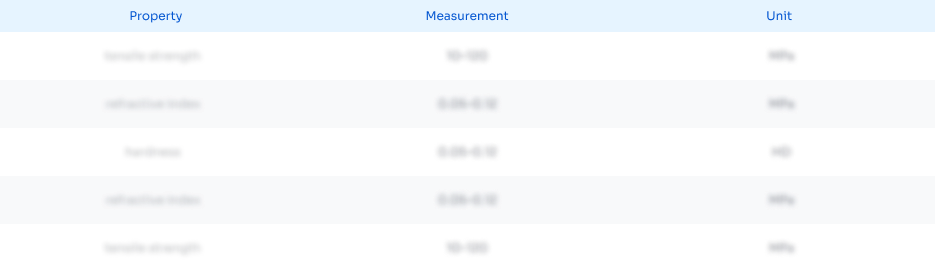
Abstract
Description
Claims
Application Information
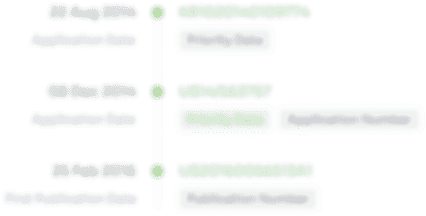
- Generate Ideas
- Intellectual Property
- Life Sciences
- Materials
- Tech Scout
- Unparalleled Data Quality
- Higher Quality Content
- 60% Fewer Hallucinations
Browse by: Latest US Patents, China's latest patents, Technical Efficacy Thesaurus, Application Domain, Technology Topic, Popular Technical Reports.
© 2025 PatSnap. All rights reserved.Legal|Privacy policy|Modern Slavery Act Transparency Statement|Sitemap|About US| Contact US: help@patsnap.com