A Cross-Modal Retrieval Method Based on Multi-layer Semantic Alignment
A cross-modal and semantic technology, applied in the field of cross-modal retrieval, can solve the problems of ignoring fine-grained image areas and text word relationships, and image features are highly dependent on detection, so as to make up for inaccurate detection, improve retrieval accuracy, and better Associated Effects
- Summary
- Abstract
- Description
- Claims
- Application Information
AI Technical Summary
Problems solved by technology
Method used
Image
Examples
Embodiment
[0120] 1. Experimental method
[0121] This experiment is run on an NVIDIA 1080Ti GPU workstation. Experiments are carried out on two public datasets, Flickr30k and MSCOCO. Each picture in the dataset corresponds to five associated sentences. The data information is shown in Table 1. Since the data set only contains two modal data, image and text, this method verifies the mutual retrieval of text and image. In the experiment, 36 regions and 2048-dimensional features were extracted from each image, and the data dimensionality was reduced to 1024 common spaces through the fully connected layer. For each sentence, the word embedding size is set to 300, sentences with insufficient length are padded with zeros, the sentence words are encoded using Bi-GRU, and the hidden unit dimension is 1024.
[0122] Table 1 Details of Flickr30k and MSCOCO datasets
[0123]
[0124] In this paper, R@K is used to evaluate the method. R@K indicates the correct query percentage among the K ret...
PUM
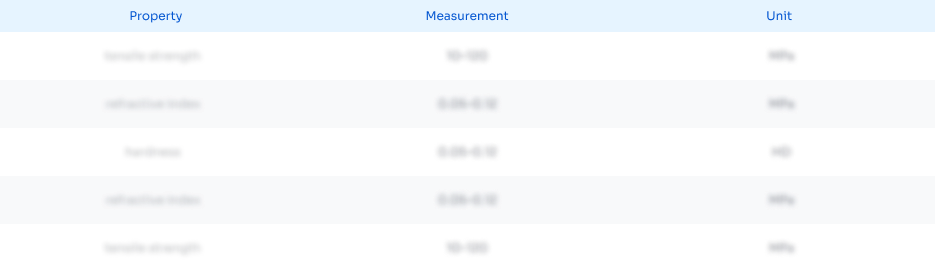
Abstract
Description
Claims
Application Information
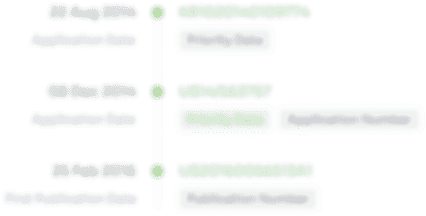
- R&D Engineer
- R&D Manager
- IP Professional
- Industry Leading Data Capabilities
- Powerful AI technology
- Patent DNA Extraction
Browse by: Latest US Patents, China's latest patents, Technical Efficacy Thesaurus, Application Domain, Technology Topic, Popular Technical Reports.
© 2024 PatSnap. All rights reserved.Legal|Privacy policy|Modern Slavery Act Transparency Statement|Sitemap|About US| Contact US: help@patsnap.com