Short-term cluster wind power prediction method based on VMD-EMD-WT signal decomposition and SDAE deep learning model
A VMD-EMD-WT, wind power prediction technology, applied in the identification of patterns in signals, power generation prediction in AC networks, wind power generation, etc. It can not meet the problems of power grid dispatching, and achieve the effect of being beneficial to economic dispatching and safe and reliable operation, reducing the curtailment rate of wind curtailment, and improving the ability of wind power to connect to the grid.
- Summary
- Abstract
- Description
- Claims
- Application Information
AI Technical Summary
Problems solved by technology
Method used
Image
Examples
Embodiment Construction
[0019] The following is a specific description in conjunction with the accompanying drawings in the embodiments of the present invention. The purpose of the present invention is to provide a VMD-EMD-WT signal decomposition technology and SDAE based on multiple integrated learning models to improve the prediction accuracy of cluster wind power generation power. Short-term cluster wind power forecasting method based on multiple integration of deep learning models.
[0020] like figure 1 As shown, a short-term cluster wind power forecasting method based on multiple integration of VMD-EMD-WT signal decomposition technology and SDAE deep learning model. This method is divided into five steps to achieve short-term cluster wind power forecasting, including the following steps:
[0021] Step 1, preprocessing the multi-dimensional NWP data and wind farm historical power data in the original feature database;
[0022] Step 2, divide the preprocessed data set into training set and test ...
PUM
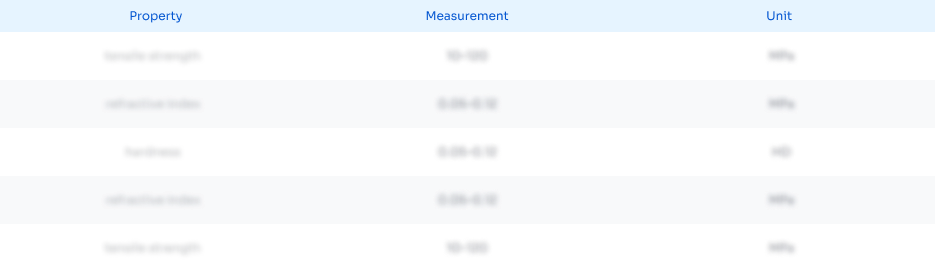
Abstract
Description
Claims
Application Information
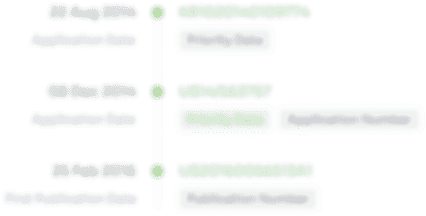
- R&D Engineer
- R&D Manager
- IP Professional
- Industry Leading Data Capabilities
- Powerful AI technology
- Patent DNA Extraction
Browse by: Latest US Patents, China's latest patents, Technical Efficacy Thesaurus, Application Domain, Technology Topic, Popular Technical Reports.
© 2024 PatSnap. All rights reserved.Legal|Privacy policy|Modern Slavery Act Transparency Statement|Sitemap|About US| Contact US: help@patsnap.com