WOA-LSTM-MC-based hydrological time series prediction optimization method
A hydrological time series, forecast optimization technology, applied in forecasting, neural learning methods, data processing applications, etc., can solve the problems of small sample set model accuracy, time-consuming training, large errors, etc., to ensure global search capabilities and local The effect of exploring ability and speeding up the convergence speed
- Summary
- Abstract
- Description
- Claims
- Application Information
AI Technical Summary
Problems solved by technology
Method used
Image
Examples
Embodiment 1
[0067]Example 1: This example is predicted on the Hankou Hydrology Station of the Yangtze River Basin, such asFigure 5 withFigure 6 As shown, including the following steps:
[0068]Step S1, the data set of this embodiment selects the hourly traffic data of Hankou Hydrology, January 1, 2019, from January 1, 2020, of which 8:00 to 2020, January 2019 5 At 7:00 on the 15th, 12,000 data were taken as a training set, and from 8:00 on June 12, 2020, 4351 data were 4351 data as a test set.
[0069]Step S11, the present embodiment sets the traffic data of the Hankou Hydrology as a forecasting factor, and sets a 1-24-hour foreseeable period, and different predictive models are established for different foreseevelopments. By correlation analysis, the first T is selected1Hourly prediction T2Hours, T1Get through correlation analysis, T2For the foresee period.
[0070]Step S21, the present embodiment takes the flow data of Hankou Station in the Yangtze River Basin as an example, and the adaptivity value i...
PUM
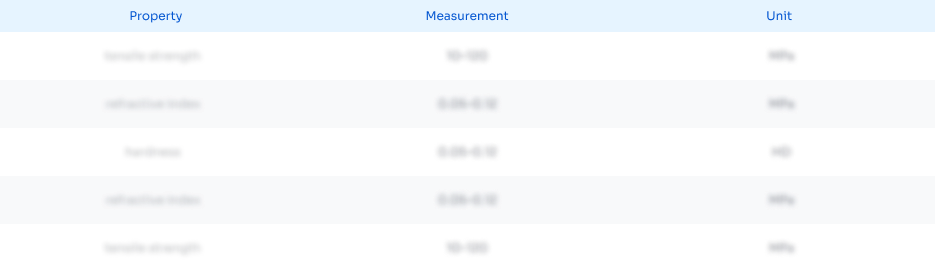
Abstract
Description
Claims
Application Information
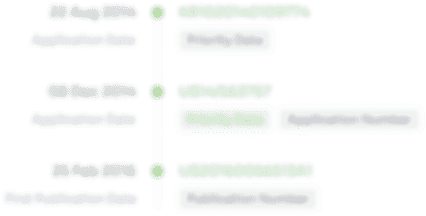
- R&D Engineer
- R&D Manager
- IP Professional
- Industry Leading Data Capabilities
- Powerful AI technology
- Patent DNA Extraction
Browse by: Latest US Patents, China's latest patents, Technical Efficacy Thesaurus, Application Domain, Technology Topic, Popular Technical Reports.
© 2024 PatSnap. All rights reserved.Legal|Privacy policy|Modern Slavery Act Transparency Statement|Sitemap|About US| Contact US: help@patsnap.com