Reinforcement learning battle game AI training method based on information bottleneck theory
A technology of reinforcement learning and information bottleneck, which is applied in the field of game intelligent AI learning, can solve problems such as single routine, reduce mutual information, and lack of flexibility in fighting between players, so as to save training time, speed up training, and improve sampling efficiency effect
- Summary
- Abstract
- Description
- Claims
- Application Information
AI Technical Summary
Problems solved by technology
Method used
Image
Examples
Embodiment
[0051] Such as figure 1 As shown, the present invention provides a kind of reinforcement learning battle game AI training method based on information bottleneck theory, comprises the following steps:
[0052] 1) Initialize the network parameters and hyperparameters of the AI training model (the CNN model is used in this example, and the specific model structure is as follows image 3 shown), set the learning rate and the number of samples sampled from the parameter distribution;
[0053] 2) Make decision-making interactions in the simulation environment through AI to obtain sample training batch data sets;
[0054] 3) Based on the sample training batch data set obtained from the interaction between AI and the environment, the reinforcement learning algorithm (A2C algorithm is used in this example) is used to iteratively train the AI training model, and the model parameters are saved in stages;
[0055] 4) Fix some parameters of the saved models at different stages, and u...
PUM
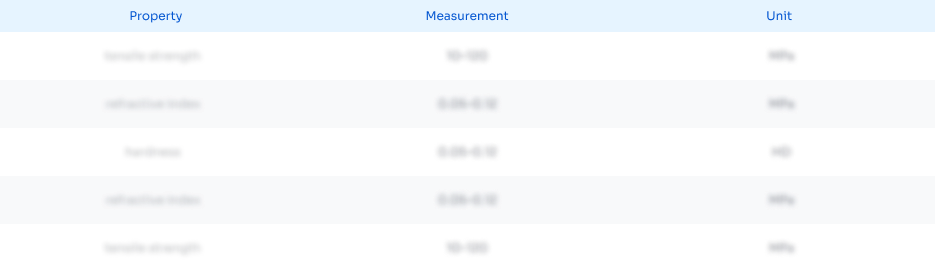
Abstract
Description
Claims
Application Information
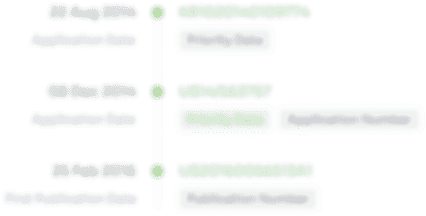
- R&D Engineer
- R&D Manager
- IP Professional
- Industry Leading Data Capabilities
- Powerful AI technology
- Patent DNA Extraction
Browse by: Latest US Patents, China's latest patents, Technical Efficacy Thesaurus, Application Domain, Technology Topic, Popular Technical Reports.
© 2024 PatSnap. All rights reserved.Legal|Privacy policy|Modern Slavery Act Transparency Statement|Sitemap|About US| Contact US: help@patsnap.com