A generative dialogue summarization method incorporating common sense knowledge
A generative, knowledge-based technology, applied in biological neural network models, instruments, computing, etc., to solve problems such as low abstraction and inaccurate dialogue summaries
- Summary
- Abstract
- Description
- Claims
- Application Information
AI Technical Summary
Problems solved by technology
Method used
Image
Examples
specific Embodiment approach 1
[0032] Embodiment 1: This embodiment is a method for generating dialogue summarization incorporating common sense knowledge, including:
[0033] Step 1: Obtain the large-scale commonsense knowledge base ConceptNet and the dialogue summary dataset SAMSum.
[0034] Step 11. Obtain ConceptNet, a large-scale common sense knowledge base:
[0035] Obtain the large-scale common sense knowledge base ConceptNet from http: / / conceptnet.io / ; the common sense knowledge contained in it exists in the form of tuples, that is, tuple knowledge, which can be expressed as:
[0036] R=(h,r,t,w),
[0037] Among them, R represents a tuple of knowledge; h represents the head entity; r represents the relationship; t represents the tail entity; w represents the weight, which represents the confidence of the relationship; knowledge R represents that the head entity h and the tail entity t have the relationship r, and the weight It is w; for example, R=(call, related, contact, 10, indicating that the r...
specific Embodiment approach 2
[0046] Embodiment 2: This embodiment is different from Embodiment 1 in that step 2 uses the acquired large-scale common sense knowledge base ConceptNet to introduce tuple knowledge into the dialogue summary data set SAMSum, and constructs a heterogeneous dialogue graph; The process is:
[0047] Step 21: Obtaining the relevant knowledge of the dialogue; for a dialogue, the present invention first obtains a series of relevant tuple knowledge from ConceptNet according to the words in the dialogue, excludes the noise knowledge, and finally obtains the tuple knowledge set related to the given dialogue, like Figure 4 ;
[0048] Step 22. Build a sentence-knowledge graph:
[0049] For the related tuple knowledge obtained in step 21, suppose there are sentence A and sentence B, word a belongs to sentence A, and word b belongs to sentence B, if the tail entity h of the related knowledge of a and b is consistent, then sentence A and sentence B connected to tail entity h; get sentence...
specific Embodiment approach 3
[0058] Embodiment 3: The difference between this embodiment and Embodiment 1 or 2 is that in step 31, a node encoder is constructed, and a bidirectional long-short-term neural network (Bi-LSTM) is used to obtain a node initialization representation and the word initialization representation The specific process is:
[0059] For the heterogeneous dialogue graph proposed by the present invention in step 2, each node v i contains|v i | words, the word sequence is where w i,n represents node v i the nth word of , n∈[1,|v i |]; use a bidirectional long-short-term neural network (Bi-LSTM) to Generate the forward hidden layer sequence and the backward hidden layer sequence Among them, the forward hidden layer state Backward Hidden State x n means w i,n The word vector representation of ; the initial representation of the node is obtained by splicing the last hidden layer representation of the forward hidden layer state with the first hidden layer representation ...
PUM
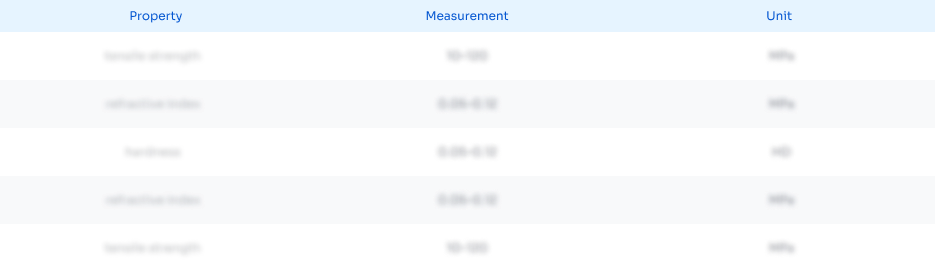
Abstract
Description
Claims
Application Information
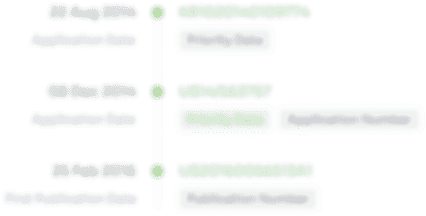
- Generate Ideas
- Intellectual Property
- Life Sciences
- Materials
- Tech Scout
- Unparalleled Data Quality
- Higher Quality Content
- 60% Fewer Hallucinations
Browse by: Latest US Patents, China's latest patents, Technical Efficacy Thesaurus, Application Domain, Technology Topic, Popular Technical Reports.
© 2025 PatSnap. All rights reserved.Legal|Privacy policy|Modern Slavery Act Transparency Statement|Sitemap|About US| Contact US: help@patsnap.com