Crowd counting model based on structured knowledge distillation and method thereof
A technology of structured knowledge and crowd counting, applied in the field of crowd counting model based on structured knowledge distillation, which can solve the problems of tedious hyperparameter adjustment, low efficiency and large computational cost.
- Summary
- Abstract
- Description
- Claims
- Application Information
AI Technical Summary
Problems solved by technology
Method used
Image
Examples
Embodiment Construction
[0049] The implementation of the present invention is described below through specific examples and in conjunction with the accompanying drawings, and those skilled in the art can easily understand other advantages and effects of the present invention from the content disclosed in this specification. The present invention can also be implemented or applied through other different specific examples, and various modifications and changes can be made to the details in this specification based on different viewpoints and applications without departing from the spirit of the present invention.
[0050] figure 1 It is a system architecture diagram of a crowd counting model based on structured knowledge distillation in the present invention. Such as figure 1 As shown, the present invention is a crowd counting model based on structured knowledge distillation, including:
[0051] The preprocessing unit 101 is configured to acquire the crowd image, and use the labeled information to g...
PUM
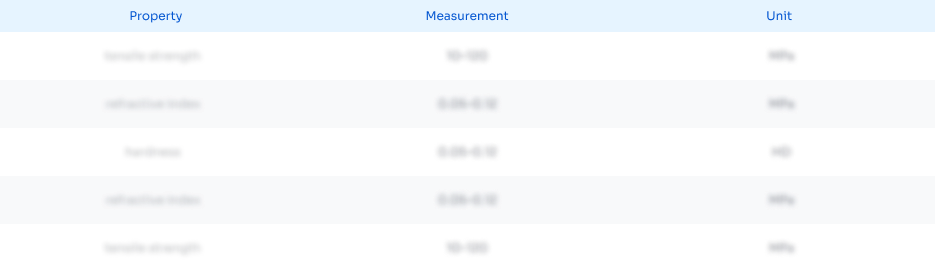
Abstract
Description
Claims
Application Information
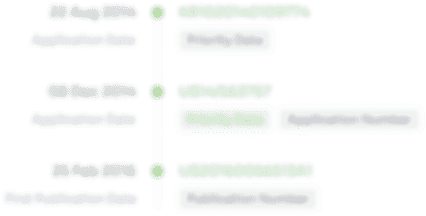
- Generate Ideas
- Intellectual Property
- Life Sciences
- Materials
- Tech Scout
- Unparalleled Data Quality
- Higher Quality Content
- 60% Fewer Hallucinations
Browse by: Latest US Patents, China's latest patents, Technical Efficacy Thesaurus, Application Domain, Technology Topic, Popular Technical Reports.
© 2025 PatSnap. All rights reserved.Legal|Privacy policy|Modern Slavery Act Transparency Statement|Sitemap|About US| Contact US: help@patsnap.com