A UAV Image Annotation Method Aided by Deep Learning Target Detection Algorithm
A target detection algorithm and deep learning technology, applied in the field of UAV image labeling assisted by deep learning target detection algorithm, to achieve the effect of large speed advantage, reduce labor cost requirements, and enhance labeling accuracy
- Summary
- Abstract
- Description
- Claims
- Application Information
AI Technical Summary
Problems solved by technology
Method used
Image
Examples
Embodiment Construction
[0026] The technical solutions of the present invention will be further described in detail below in conjunction with the accompanying drawings and embodiments.
[0027] The present invention provides a UAV image tagging method assisted by a deep learning target detection algorithm. The data sets are grouped according to the number of targets to be marked, and then the target detection depth is updated sequentially by using the training set with the number of targets to be marked from small to large. The network, on the one hand, saves labor costs as much as possible, and on the other hand, it can obtain more accurate UAV image annotations. The present invention specifically includes two cases of marking the whole picture of the UAV image and marking the partial area of the UAV image, such as figure 1 As shown in FIG. 2 , it is an overall implementation process, and the implementation steps in the two cases are described below.
[0028] In the case of full-scale annotation ...
PUM
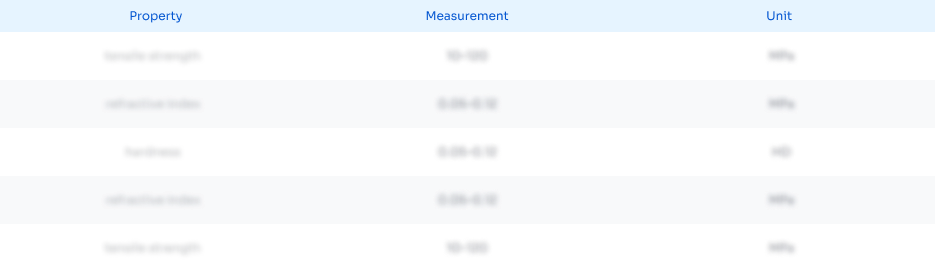
Abstract
Description
Claims
Application Information
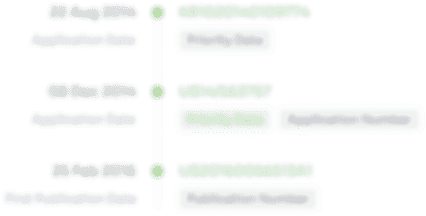
- Generate Ideas
- Intellectual Property
- Life Sciences
- Materials
- Tech Scout
- Unparalleled Data Quality
- Higher Quality Content
- 60% Fewer Hallucinations
Browse by: Latest US Patents, China's latest patents, Technical Efficacy Thesaurus, Application Domain, Technology Topic, Popular Technical Reports.
© 2025 PatSnap. All rights reserved.Legal|Privacy policy|Modern Slavery Act Transparency Statement|Sitemap|About US| Contact US: help@patsnap.com