Unsupervised monocular depth estimation algorithm based on deep learning
A technology of depth estimation and deep learning, applied in neural learning methods, computing, computer components, etc., can solve problems such as the impact of prediction accuracy
- Summary
- Abstract
- Description
- Claims
- Application Information
AI Technical Summary
Problems solved by technology
Method used
Image
Examples
Embodiment Construction
[0057] The technical solution of the present invention will be further described in conjunction with the accompanying drawings and embodiments.
[0058] see figure 1 , the algorithm model of the present invention consists of three parts: depth network DepthNet, camera pose network PoseNet and optical flow network FlowNet. The depth network DepthNet outputs a depth image with the same resolution as the monocular input image, and the depth value is expressed in grayscale. The camera pose network PoseNet is used to estimate the pose transformation of the camera in three-dimensional space between adjacent frame images, and the light The flow network FlowNet is used to estimate the full optical flow between adjacent frame images, figure 2 It is the generation confrontation network structure of the optical flow network FlowNet.
[0059] Based on the above model, the present invention designs an unsupervised monocular depth estimation algorithm based on deep learning. By comparing...
PUM
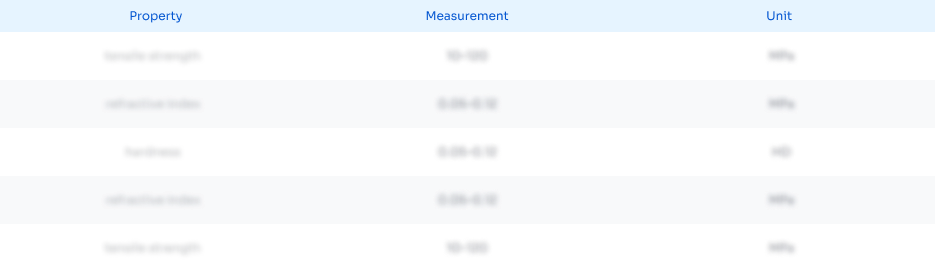
Abstract
Description
Claims
Application Information
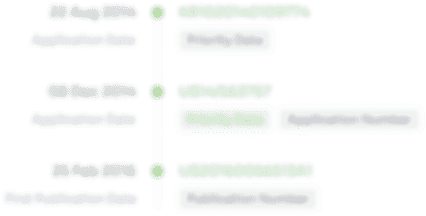
- R&D
- Intellectual Property
- Life Sciences
- Materials
- Tech Scout
- Unparalleled Data Quality
- Higher Quality Content
- 60% Fewer Hallucinations
Browse by: Latest US Patents, China's latest patents, Technical Efficacy Thesaurus, Application Domain, Technology Topic, Popular Technical Reports.
© 2025 PatSnap. All rights reserved.Legal|Privacy policy|Modern Slavery Act Transparency Statement|Sitemap|About US| Contact US: help@patsnap.com