Human face image super-resolution recognition method based on fractional order multi-set partial least squares
A partial least squares, face image technology, applied in the field of face super-resolution recognition, can solve the problem of not being able to learn the correlation of multiple resolutions at the same time, covariance matrix deviation, etc., to improve processing efficiency and face The effect of recognition rate
- Summary
- Abstract
- Description
- Claims
- Application Information
AI Technical Summary
Problems solved by technology
Method used
Image
Examples
Embodiment Construction
[0041] Such as figure 1 A kind of face image super-resolution recognition method based on fractional order multi-set partial least squares shown, comprises the following steps:
[0042] Step 1 In the training phase, use the training set to learn the correlation between different resolution views, use PCA to reduce the image dimension, use the fractional order idea to re-estimate the intra-group and inter-group covariance matrix, calculate the FMPLS projection matrix, and convert the principal component features Projected onto the consistent coherent subspace of FMPLS;
[0043] (1) For m multidimensional centralized random variables where d i means x i The dimension of FMPLS aims to solve the following optimization problem to find a set of linear transformations
[0044]
[0045] where Tr(·) represents the trace of the matrix, is the fractionally reestimated covariance matrix:
[0046]
[0047] (2) For re-estimating the covariance matrix using fractional order, us...
PUM
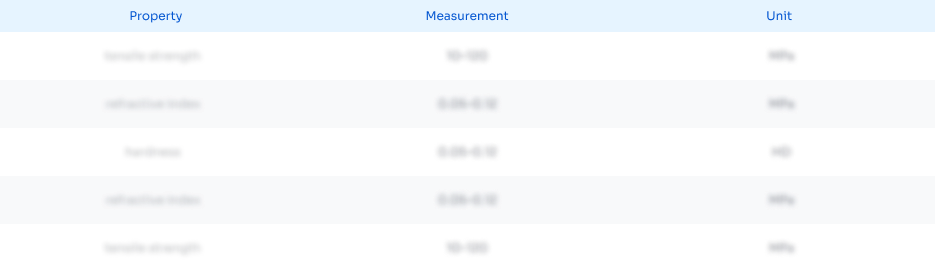
Abstract
Description
Claims
Application Information
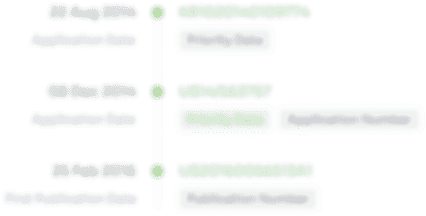
- R&D Engineer
- R&D Manager
- IP Professional
- Industry Leading Data Capabilities
- Powerful AI technology
- Patent DNA Extraction
Browse by: Latest US Patents, China's latest patents, Technical Efficacy Thesaurus, Application Domain, Technology Topic, Popular Technical Reports.
© 2024 PatSnap. All rights reserved.Legal|Privacy policy|Modern Slavery Act Transparency Statement|Sitemap|About US| Contact US: help@patsnap.com