Deep reinforcement learning robot control method based on priority experience playback
A technology of intensive learning and prioritization, applied in the direction of comprehensive factory control, program control manipulator, manipulator, etc., to achieve good operational performance, improve learning efficiency and effect
- Summary
- Abstract
- Description
- Claims
- Application Information
AI Technical Summary
Problems solved by technology
Method used
Image
Examples
Embodiment Construction
[0027] The overall flow chart of the algorithm is as follows figure 1 shown. The details will be described below.
[0028]The priority experience playback technology method based on the state change of the target object described in this embodiment is to speed up the learning speed and improve the learning effect through the way of priority experience playback during the interactive learning process between the robot and the environment, including the following steps :
[0029] S1. Construct a virtualization environment, and complete the initialization setting of the virtual environment.
[0030] In this embodiment, the present invention is based on a virtualized environment, and the training is completed in the virtualized environment.
[0031] The virtual environment is a simulation environment based on the real environment and robots, and mainly includes two parts: the simulated task environment and the simulated robot. The virtual environment is built based on GYM, and...
PUM
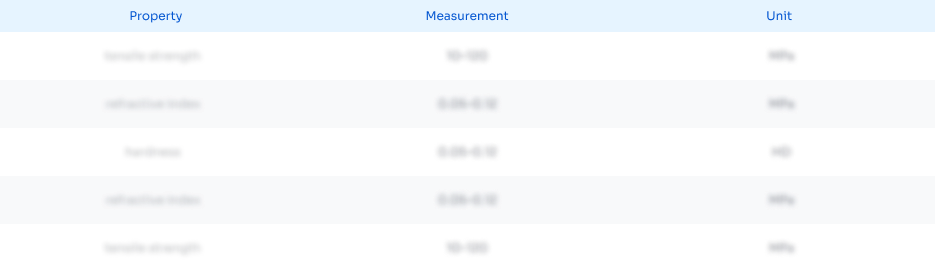
Abstract
Description
Claims
Application Information
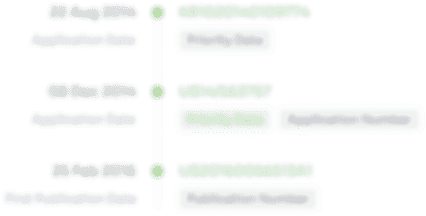
- R&D Engineer
- R&D Manager
- IP Professional
- Industry Leading Data Capabilities
- Powerful AI technology
- Patent DNA Extraction
Browse by: Latest US Patents, China's latest patents, Technical Efficacy Thesaurus, Application Domain, Technology Topic.
© 2024 PatSnap. All rights reserved.Legal|Privacy policy|Modern Slavery Act Transparency Statement|Sitemap