Subject term embedding disambiguation method and system based on LDA
A subject word and subject technology, applied in the field of LDA-based subject word embedding and disambiguation, can solve problems such as polysemy, different word meanings, and difficulty in capturing word meanings, and achieve obvious performance improvement and rich semantic information.
- Summary
- Abstract
- Description
- Claims
- Application Information
AI Technical Summary
Problems solved by technology
Method used
Image
Examples
Embodiment 1
[0048] Embodiment 1: as figure 1 As shown, an LDA-based keyword embedding disambiguation method, the specific steps are:
[0049] Step1: Based on the large-scale Wiki corpus without word meaning annotation, use the online LDA algorithm to train the topic model;
[0050]Step2: Based on the topic model, classify each document of the Wiki corpus into each topic to form various topic document sets, and then use Word2Vec to train the word vector under each topic for each topic document set, which is the topic word vector;
[0051] Step3: Based on the small-scale SemCor corpus with semantic annotations, use the topic model and the topic word vector to calculate the context vector;
[0052] Step4: Concatenate the context vector and other traditional semantic features, use SVM to train and test the disambiguation model.
[0053] Further, the Step1 is specifically:
[0054] Step1.1: Do word segmentation for the Wiki corpus, remove the non-word symbols in each document for word segm...
Embodiment 2
[0079] Embodiment 2: a kind of LDA-based keyword embedding disambiguation method, comprising:
[0080] Topic model training steps:
[0081] Step1.1: Do word segmentation for the Wiki corpus, remove the non-word symbols in each document for word segmentation, and convert it into a document with one line;
[0082] Step1.2: Then use WordNet to restore the lemmatization of the corpus;
[0083] Step1.3: Then use the preset stop word set to remove all stop words in the corpus and generate a new Wiki corpus;
[0084] Step1.4: Finally, based on the Wiki corpus, use online LDA to train the topic model, including the document-topic probability distribution p(t i |d) and word-topic probability distribution p(t j |w). Among them, d represents the current document, w represents the current word, and t i represents the ith topic.
[0085] Example:
[0086] Suppose there is a corpus containing three documents: {"Anarchism draws on many currents of thought and strategy.", "Anarchism do...
PUM
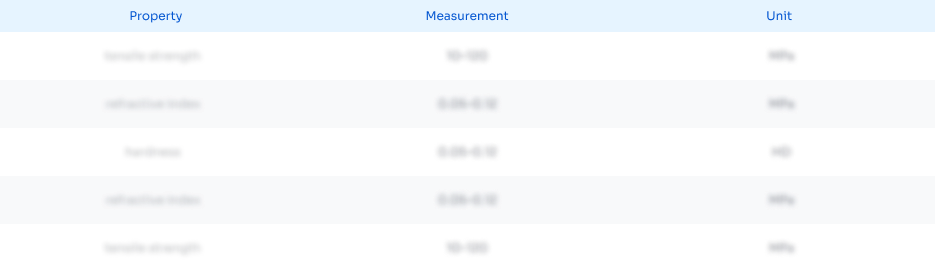
Abstract
Description
Claims
Application Information
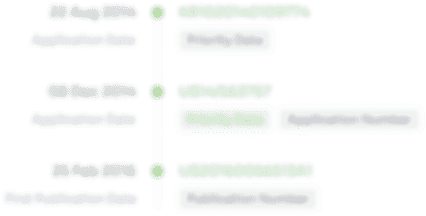
- R&D Engineer
- R&D Manager
- IP Professional
- Industry Leading Data Capabilities
- Powerful AI technology
- Patent DNA Extraction
Browse by: Latest US Patents, China's latest patents, Technical Efficacy Thesaurus, Application Domain, Technology Topic, Popular Technical Reports.
© 2024 PatSnap. All rights reserved.Legal|Privacy policy|Modern Slavery Act Transparency Statement|Sitemap|About US| Contact US: help@patsnap.com