Learning and reasoning method of hybrid Bayesian network
A Bayesian network and inference method technology, applied in the field of anomaly detection and localization of Bayesian networks, can solve problems such as insufficient accuracy and timeliness, achieve good accuracy and timeliness, simplify parameter learning steps, and ensure The effect of computational efficiency
- Summary
- Abstract
- Description
- Claims
- Application Information
AI Technical Summary
Problems solved by technology
Method used
Image
Examples
Embodiment 1
[0058] This embodiment provides a learning and reasoning method for a hybrid Bayesian network, such as figure 1 As shown, the learning and reasoning method includes the following steps:
[0059] S1: Use the supervised top-down discretization algorithm CACC (Class-Stribbute Contingency Coefficient) to discretize continuous variables;
[0060] S2: Gibbs Sampling (Gibbs sampling) algorithm is used to solve the parameter learning problem of incomplete data sets, and a complete hybrid Bayesian network model is obtained;
[0061] S3: Using the Markov chain Monte Carlo algorithm (MCMC) and the joint tree algorithm as a hybrid Bayesian network reasoning algorithm to seek a reasoning algorithm that is more suitable for the application scenario.
[0062] After the present invention builds the hybrid Bayesian network model, according to different scenarios, according to needs, select Markov chain Monte Carlo algorithm (MCMC) or joint tree algorithm, reason it, and then get "abnormal det...
PUM
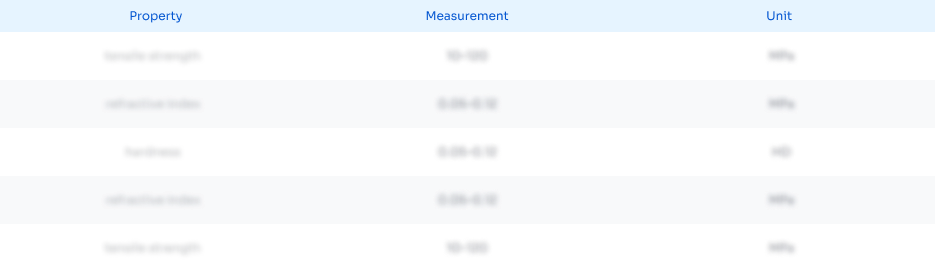
Abstract
Description
Claims
Application Information
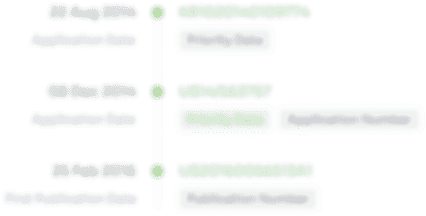
- R&D
- Intellectual Property
- Life Sciences
- Materials
- Tech Scout
- Unparalleled Data Quality
- Higher Quality Content
- 60% Fewer Hallucinations
Browse by: Latest US Patents, China's latest patents, Technical Efficacy Thesaurus, Application Domain, Technology Topic, Popular Technical Reports.
© 2025 PatSnap. All rights reserved.Legal|Privacy policy|Modern Slavery Act Transparency Statement|Sitemap|About US| Contact US: help@patsnap.com