A network structure deanonymization system and method based on matrix decomposition
A technology of network structure and matrix decomposition, applied in transmission systems, digital transmission systems, data exchange networks, etc., can solve problems such as limited accuracy and lack of global structural information
- Summary
- Abstract
- Description
- Claims
- Application Information
AI Technical Summary
Problems solved by technology
Method used
Image
Examples
Embodiment 1
[0044] In the present embodiment, the present invention proposes a matrix-based network structure to anonymization system, including a dynamic network acquisition data module, a low rank sparse model training module, a construction module, an optimization module of a non-negative matrix decomposition training model, and an optimization module. in:
[0045] Dynamic Network-Static Network Conversion Module for accessing static networks and static networks in a static network and a static network adjacency matrix according to social network data of real-time dynamic network;
[0046] Low rank sparse model training module, used to constrain the local feature structure of each static network while removing noise of each static network;
[0047] The construction module of non-negative matrix decomposition training model is used to capture the intrinsic rules of the network, and portray the potential features of the network;
[0048] Optimization modules for reducing the randomness of th...
Embodiment 2
[0065] This embodiment proposes a matrix-based network structure to go anonymization method, such as figure 1 , Includes the following steps:
[0066] S1, obtain data sources, data sources include the topology structure between the various networks and the topology structure between the relationship between users in the network; figure 2 The dynamic network shown, for example, the friend relationship topology on Tencent Weibo may be similar to the friend relationship topology on Facebook. The two networks have a part of the common user, the relationship between the network, and the network between users. The topology of the relationship is the topological structure of the relationship between users in a network;
[0067] S2, such as figure 2 , The data set of the dynamic social network is divided into T-static network data set according to the time, and the adjacent matrix corresponding to each static network structure information is obtained;
[0068] S3, the adjacent matrix inpu...
Embodiment 3
[0085] As an alternative embodiment, the solving process of the target matrix includes:
[0086]
[0087] Among them, Rank (S t ), Λ represent damping coefficient; e t A noise error matrix of the TF; A t Represents the neighboring matrix of the tip of the tip; t Represents the target matrix of the Type T static network; || E t || 0 Indicates the sparse noise constraint; s represents the target matrix after training after a low rare model; E represents the noise error matrix of the network.
PUM
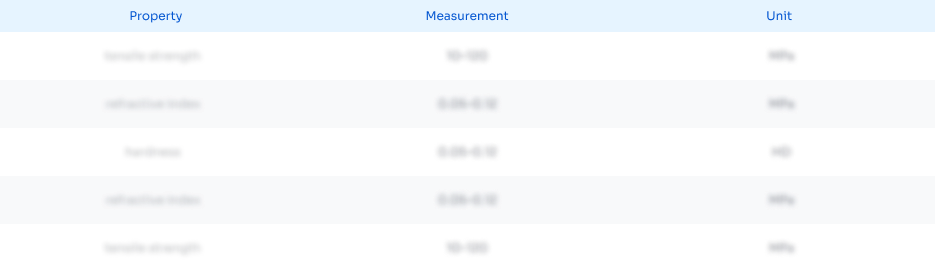
Abstract
Description
Claims
Application Information
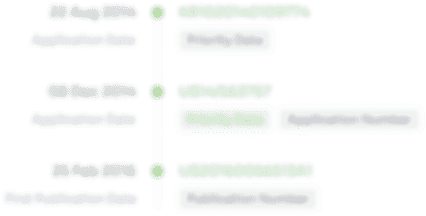
- R&D
- Intellectual Property
- Life Sciences
- Materials
- Tech Scout
- Unparalleled Data Quality
- Higher Quality Content
- 60% Fewer Hallucinations
Browse by: Latest US Patents, China's latest patents, Technical Efficacy Thesaurus, Application Domain, Technology Topic, Popular Technical Reports.
© 2025 PatSnap. All rights reserved.Legal|Privacy policy|Modern Slavery Act Transparency Statement|Sitemap|About US| Contact US: help@patsnap.com