Rolling bearing residual life prediction method based on convolutional long-short-term memory recurrent neural network
A cyclic neural network, long and short-term memory technology, applied in biological neural network models, neural architectures, instruments, etc., can solve problems such as increasing computational complexity and large errors in prediction results, and achieve effective feature extraction, simplified operations, and predictions. accurate effect
- Summary
- Abstract
- Description
- Claims
- Application Information
AI Technical Summary
Problems solved by technology
Method used
Image
Examples
Embodiment Construction
[0030] Exemplary embodiments of the present disclosure will be described in more detail below with reference to the accompanying drawings. Although exemplary embodiments of the present disclosure are shown in the drawings, it should be understood that the present disclosure may be embodied in various forms and should not be limited by the embodiments set forth herein. Rather, these embodiments are provided for more thorough understanding of the present disclosure and to fully convey the scope of the present disclosure to those skilled in the art.
[0031] Such as figure 1 As shown, the method for predicting the remaining life of rolling bearings based on the convolutional long-short-term memory recurrent neural network provided by the present invention includes:
[0032] Obtain the vibration acceleration signal of the rolling bearing as a data sample.
[0033] Establish a convolutional autoencoder, use data samples as input, and train to obtain a multi-layer convolutional au...
PUM
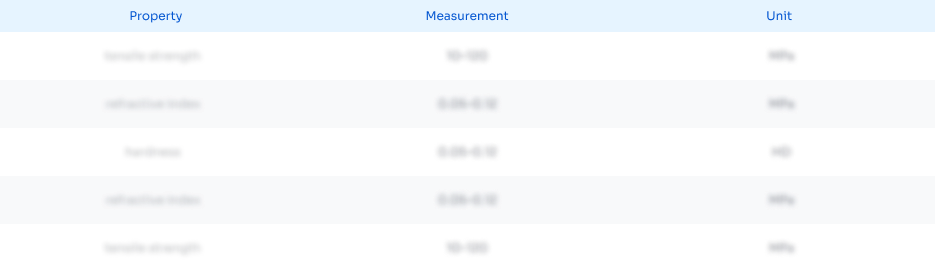
Abstract
Description
Claims
Application Information
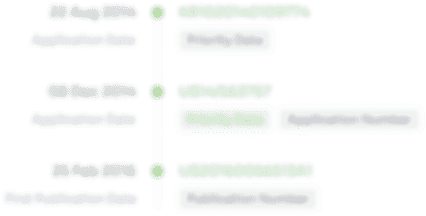
- Generate Ideas
- Intellectual Property
- Life Sciences
- Materials
- Tech Scout
- Unparalleled Data Quality
- Higher Quality Content
- 60% Fewer Hallucinations
Browse by: Latest US Patents, China's latest patents, Technical Efficacy Thesaurus, Application Domain, Technology Topic, Popular Technical Reports.
© 2025 PatSnap. All rights reserved.Legal|Privacy policy|Modern Slavery Act Transparency Statement|Sitemap|About US| Contact US: help@patsnap.com