Chemical production process monitoring method based on multi-block projection non-negative matrix factorization
A non-negative matrix decomposition, chemical production technology, applied in the direction of program control, comprehensive factory control, electrical program control, etc., can solve the problems of reduced calculation speed, disadvantage, and large number of statistics.
- Summary
- Abstract
- Description
- Claims
- Application Information
AI Technical Summary
Problems solved by technology
Method used
Image
Examples
Embodiment 1
[0069] Embodiment 1: A chemical production process monitoring method based on multi-block projected non-negative matrix factorization (MPNMF), which is used to process physical quantity data obtained at multiple physical quantity monitoring points in the chemical production process to identify physical quantities related to faults Data, which is convenient for production and maintenance personnel to discover problems in chemical production early and deal with them accordingly. The physical quantity monitored by the physical quantity monitoring point includes at least one of temperature, pressure, liquid level, fluid velocity and flow rate, and the physical quantity monitoring of the TE process Refer to Table 1 for the points and corresponding monitored physical quantities, including the following steps:
[0070] Step 1: Establish an offline MPNMF model.
[0071] (1) Run the TE process simulation system, input n historical normal samples of physical quantities monitored by m ph...
experiment example 1
[0106] Experimental example 1: This experimental example uses the TE model to simulate a specific application of a chemical production process fault monitoring method based on multi-block projected non-negative matrix factorization (MPNMF). Table 1 lists the data collected by 33 physical quantity monitoring points in the TE process. Physical quantities, these physical quantities include temperature, pressure, liquid level, fluid velocity flow rate and power. Table 2 lists 21 kinds of faults that are highly related to the physical quantity data collected by these 33 physical quantity monitoring points. Table 3 lists the faults through the full link algorithm For the block results of the process physical quantity, Table 4 shows the fault monitoring accuracy rate when using NMF, PNMF, and MPNMF to monitor the 21 kinds of faults respectively ( where T 2 ,N 2 ,SPE, BIC SPE are the monitoring statistics of different methods.
[0107]Table 1 Physical quantity monitoring points ...
PUM
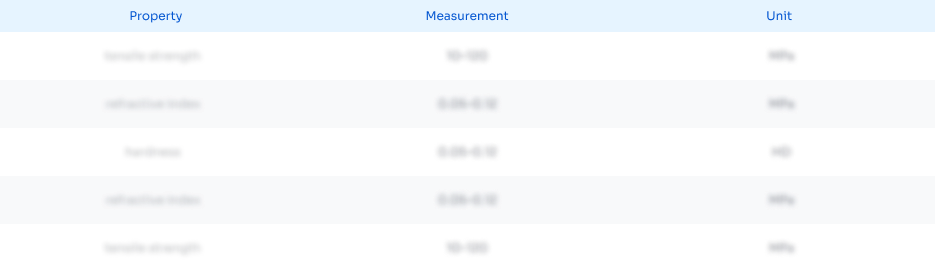
Abstract
Description
Claims
Application Information
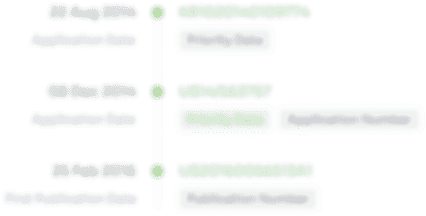
- R&D Engineer
- R&D Manager
- IP Professional
- Industry Leading Data Capabilities
- Powerful AI technology
- Patent DNA Extraction
Browse by: Latest US Patents, China's latest patents, Technical Efficacy Thesaurus, Application Domain, Technology Topic.
© 2024 PatSnap. All rights reserved.Legal|Privacy policy|Modern Slavery Act Transparency Statement|Sitemap