Reinforced learning method for dynamically optimizing logistics scheduling and path planning in machining process
A processing and reinforcement learning technology, applied in neural learning methods, logistics, biological neural network models, etc., can solve problems such as the inability to automatically adjust real-time dynamic logistics scheduling schemes, and ignoring dynamic characteristics.
- Summary
- Abstract
- Description
- Claims
- Application Information
AI Technical Summary
Problems solved by technology
Method used
Image
Examples
Embodiment Construction
[0079] In order to make the object, technical solution and advantages of the present invention clearer, the present invention will be further described in detail below in conjunction with the accompanying drawings and embodiments. It should be understood that the specific embodiments described here are only used to explain the present invention, not to limit the present invention. In addition, the technical features involved in the various embodiments of the present invention described below can be combined with each other as long as they do not constitute a conflict with each other.
[0080] Such as figure 1 , figure 2 As shown, a kind of reinforcement learning method for logistics scheduling and path planning in a dynamic optimization process of a preferred embodiment of the present invention includes the following steps:
[0081] Step 1: build a reinforcement learning decision model, the decision model accepts the total state matrix at any time as input;
[0082] The re...
PUM
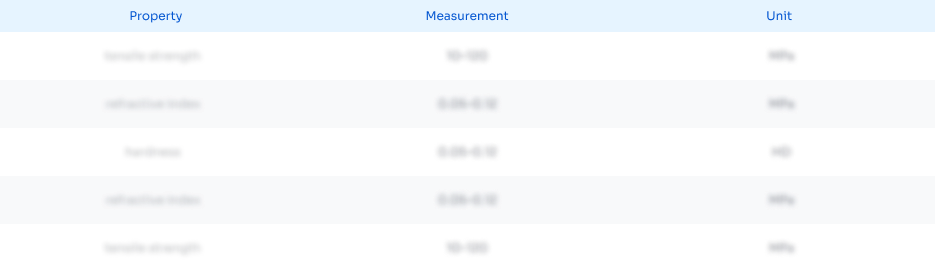
Abstract
Description
Claims
Application Information
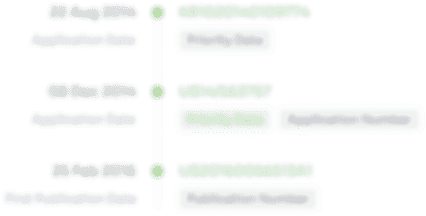
- Generate Ideas
- Intellectual Property
- Life Sciences
- Materials
- Tech Scout
- Unparalleled Data Quality
- Higher Quality Content
- 60% Fewer Hallucinations
Browse by: Latest US Patents, China's latest patents, Technical Efficacy Thesaurus, Application Domain, Technology Topic, Popular Technical Reports.
© 2025 PatSnap. All rights reserved.Legal|Privacy policy|Modern Slavery Act Transparency Statement|Sitemap|About US| Contact US: help@patsnap.com