An auxiliary system for predicting the extent of early cancer lesions based on deep learning
A deep learning and auxiliary system technology, applied in the field of image processing, can solve the problems of low direct diagnosis accuracy and prone to errors, etc., and achieve the effects of improving recognition ability, reducing workload, and significant social and economic value
- Summary
- Abstract
- Description
- Claims
- Application Information
AI Technical Summary
Problems solved by technology
Method used
Image
Examples
Embodiment 1
[0038] Such as figure 1 As shown, a deep learning-based auxiliary system for predicting the range of early cancer lesions includes an image acquisition module, a model building module, a range division module, and a display module.
[0039] The image acquisition module is used to obtain the sample image of the digestive tract endoscope with the target frame, preprocess the sample image, record the coordinate information of the end point of the target frame, sort them randomly, generate a training image set, and perform all Sample images are normalized. In this implementation, the normalization process refers to normalizing the sample image into DICOM format, NIfTI format or original binary format. The target frame is a rectangular frame, and the number of target frame end points is four.
[0040] In this embodiment, the sample image includes one or more cancer categories in early esophageal cancer, early gastric cancer, and early colon cancer, and each cancer category corres...
Embodiment 2
[0057] An auxiliary system for predicting the range of early cancer lesions based on deep learning differs from Embodiment 1 in that when the construction unit outputs a convolutional neural network model, the image acquisition module marks the adjusted preprocessing method as an effective preprocessing method. The image acquisition module is also used to acquire the images to be diagnosed from the gastrointestinal endoscope, preprocess the images to be diagnosed by an effective preprocessing method, and send the preprocessed images to be diagnosed to the range division module.
[0058] The image to be diagnosed is preprocessed through an effective preprocessing method, so that the image to be diagnosed can meet the input requirements of the convolutional neural network model, and the recognition accuracy can be improved.
Embodiment 3
[0060] An auxiliary system for predicting the range of early cancer lesions based on deep learning, the difference from Embodiment 2 is that it also includes a judgment module and an environment regulation module;
[0061] The evaluation module is used to obtain the image to be diagnosed with the target frame drawn by the doctor, compare the target frame drawn by the doctor in the same image to be diagnosed with the target frame drawn by the range division module, and judge whether they are consistent. If not, the evaluation module outputs request evaluation information ; At present, the convolutional neural network model is still mainly used for auxiliary diagnosis, and the probability of misdiagnosis is reduced through double verification, while improving the diagnostic efficiency of doctors.
[0062] The judging module is also used to receive judged information; in this embodiment, the judged information is that the doctor is correct or the convolutional neural network model...
PUM
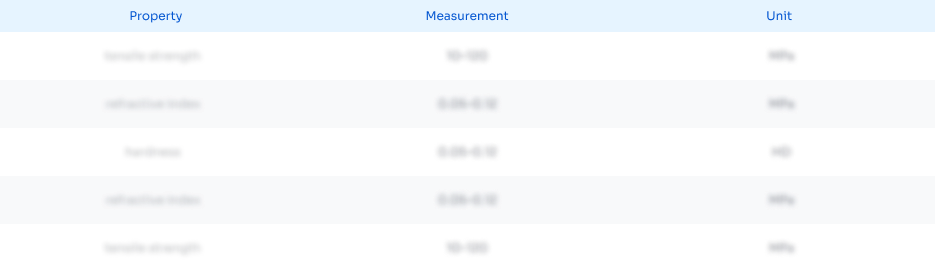
Abstract
Description
Claims
Application Information
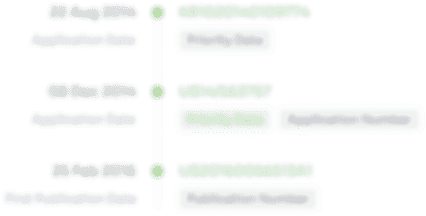
- R&D Engineer
- R&D Manager
- IP Professional
- Industry Leading Data Capabilities
- Powerful AI technology
- Patent DNA Extraction
Browse by: Latest US Patents, China's latest patents, Technical Efficacy Thesaurus, Application Domain, Technology Topic, Popular Technical Reports.
© 2024 PatSnap. All rights reserved.Legal|Privacy policy|Modern Slavery Act Transparency Statement|Sitemap|About US| Contact US: help@patsnap.com