Data classification method based on improved local abnormal factor detection
A technology of local abnormal factor and data classification, applied in the field of data processing, it can solve problems such as failure to meet expected requirements, poor stability of clustering result accuracy, and failure to take into account the correlation of data within clusters, to achieve the effect of improving accuracy.
- Summary
- Abstract
- Description
- Claims
- Application Information
AI Technical Summary
Problems solved by technology
Method used
Image
Examples
experiment example
[0086] Experimental example: prove the practicability of the inventive method, concrete steps are as follows:
[0087] Select six public data sets of Iris, Wine, Seeds, Wifi Localization, CMC, and Abalone in the UCI database, and test the results of K-means++, FCM, OFMMK-means, and optimized algorithms respectively. A detailed description of the datasets used is shown in Table 1.
[0088] Table 1 is the data set of the laboratory
[0089]
[0090] In the LOF algorithm, the parameter k_dist represents the number of detected neighborhood points. The larger the value is, the more sample points are selected, and the accuracy of clustering is more easily affected by the LOF value. This paper uses the above six data sets to do the following experiments on the value of the parameter k_dist, such as figure 1 shown.
[0091] Run the K-means++ algorithm, FCM algorithm, OFMMK-means algorithm, and the proposed optimization algorithm on the sample data sets Iris, Wine, Seeds, Wifi L...
PUM
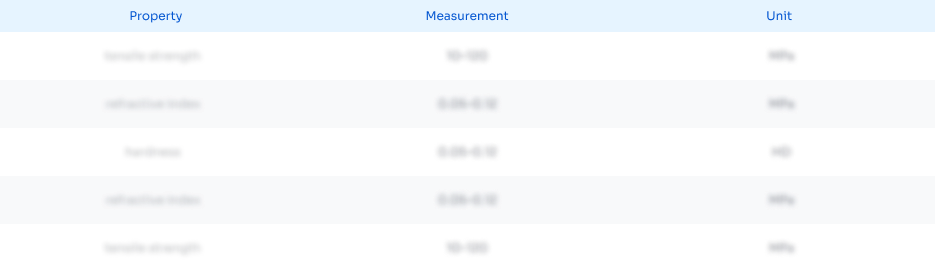
Abstract
Description
Claims
Application Information
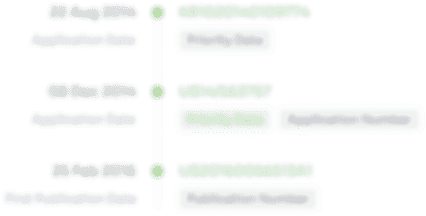
- R&D Engineer
- R&D Manager
- IP Professional
- Industry Leading Data Capabilities
- Powerful AI technology
- Patent DNA Extraction
Browse by: Latest US Patents, China's latest patents, Technical Efficacy Thesaurus, Application Domain, Technology Topic, Popular Technical Reports.
© 2024 PatSnap. All rights reserved.Legal|Privacy policy|Modern Slavery Act Transparency Statement|Sitemap|About US| Contact US: help@patsnap.com