Disease danger factor extracting method based on improved K-means clustering
A technology of risk factors and extraction methods, which is applied in the fields of big data technology and medicine, can solve problems such as difficulty, low clustering accuracy, and large amount of calculation, and achieve high accuracy and improve accuracy
- Summary
- Abstract
- Description
- Claims
- Application Information
AI Technical Summary
Problems solved by technology
Method used
Image
Examples
Embodiment
[0063] 1. Construct a user information matrix and label vector based on a user questionnaire for a certain disease: In this example, a breast cancer data set is used to construct a user information matrix and label vector. There are 569 cell biopsy cases in total, and each case has 30 breasts The characteristics of the nucleus displayed on the mass biopsy image, the answer is a numerical index, including the nucleus radius (radius), texture (texture), perimeter (perimeter), area (area), smoothness (smoothness), concavity (concavity) ), symmetry, compactness, concave points, mean, standard deviation and maximum value of fractal dimension. The size of the user data information matrix is 569*31, and the first column represents the unique identification number of the case. The problem features in this embodiment are specifically: ['mean radius', 'meantexture', 'mean perimeter', 'mean area', 'mean smoothness', 'mean compactness', 'mean concavity', 'mean concave points', 'mean sy...
PUM
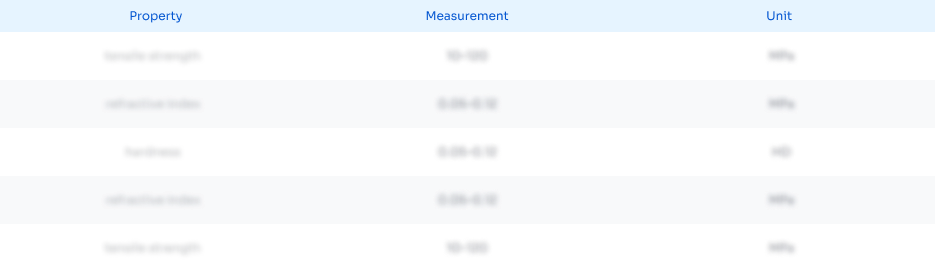
Abstract
Description
Claims
Application Information
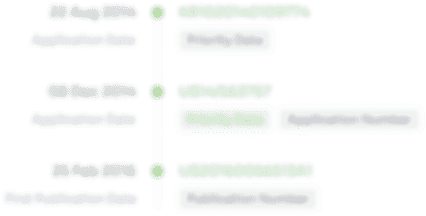
- R&D Engineer
- R&D Manager
- IP Professional
- Industry Leading Data Capabilities
- Powerful AI technology
- Patent DNA Extraction
Browse by: Latest US Patents, China's latest patents, Technical Efficacy Thesaurus, Application Domain, Technology Topic, Popular Technical Reports.
© 2024 PatSnap. All rights reserved.Legal|Privacy policy|Modern Slavery Act Transparency Statement|Sitemap|About US| Contact US: help@patsnap.com