Manifold learning network and computer vision image set classification method based on the same
A computer vision and manifold learning technology, applied in the field of manifold learning, can solve the problems of complex back-propagation calculation and many learning parameters, and achieve the effect of simple and effective training, avoiding matrix calculation, and improving classification accuracy.
- Summary
- Abstract
- Description
- Claims
- Application Information
AI Technical Summary
Benefits of technology
Problems solved by technology
Method used
Image
Examples
Embodiment Construction
[0049] The present invention will be described in detail below in conjunction with the accompanying drawings and specific embodiments. This embodiment is carried out on the premise of the technical solution of the present invention, and detailed implementation methods and specific operation processes are given, but the protection scope of the present invention is not limited to the following embodiments.
[0050] Such as figure 1 As shown, the present invention provides a manifold learning network, comprising: an input layer for obtaining a symmetric positive definite manifold corresponding to an image set to be classified; a fully connected layer for feature extraction of data received by the input layer; The correction layer is used to adjust the extracted feature values to maintain the consistency of the sample space; the Riemann pooling layer is used to perform pooling operations on features to reduce network complexity; the second logarithmic mapping layer is used to co...
PUM
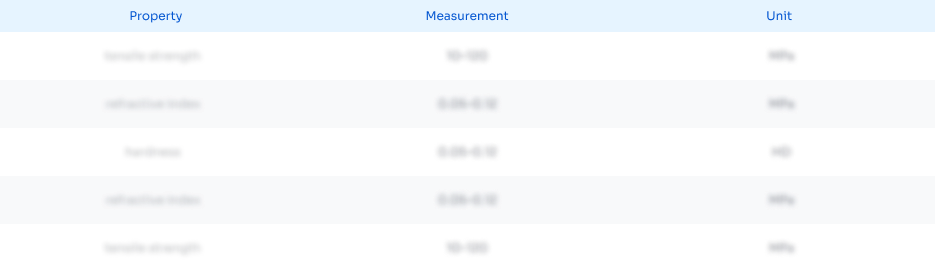
Abstract
Description
Claims
Application Information
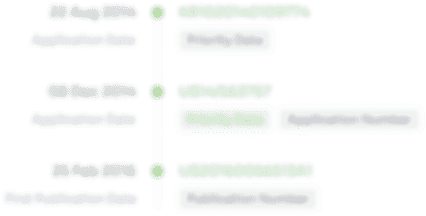
- R&D Engineer
- R&D Manager
- IP Professional
- Industry Leading Data Capabilities
- Powerful AI technology
- Patent DNA Extraction
Browse by: Latest US Patents, China's latest patents, Technical Efficacy Thesaurus, Application Domain, Technology Topic, Popular Technical Reports.
© 2024 PatSnap. All rights reserved.Legal|Privacy policy|Modern Slavery Act Transparency Statement|Sitemap|About US| Contact US: help@patsnap.com