Deep reinforcement learning-based low-speed vehicle following decision-making method
A technology that reinforces learning and decision-making methods, applied in vehicle position/route/altitude control, motor vehicles, two-dimensional position/airway control, etc., can solve problems such as gaps, improve fidelity, improve driving comfort and traffic Effects of security, strong versatility and flexibility
- Summary
- Abstract
- Description
- Claims
- Application Information
AI Technical Summary
Problems solved by technology
Method used
Image
Examples
Embodiment Construction
[0043] Below in conjunction with accompanying drawing and specific embodiment the present invention is described in further detail:
[0044] The present invention provides a vehicle low-speed car-following decision-making method based on deep reinforcement learning. The vehicle low-speed car-following decision-making method based on deep reinforcement learning not only improves driving comfort, but also ensures traffic safety, and improves the safety of congested lanes. smooth rate
[0045] In this example, if figure 1 The shown frame diagram provides the specific process of this embodiment:
[0046] Step 101, receiving the position, speed, and acceleration information of the front vehicle and the rear vehicle in real time through the Internet of Vehicles, and expressing the current state and behavior of the unmanned vehicle as the environmental state, specifically including:
[0047] (1) The position, speed, and acceleration information of the three vehicles in front receiv...
PUM
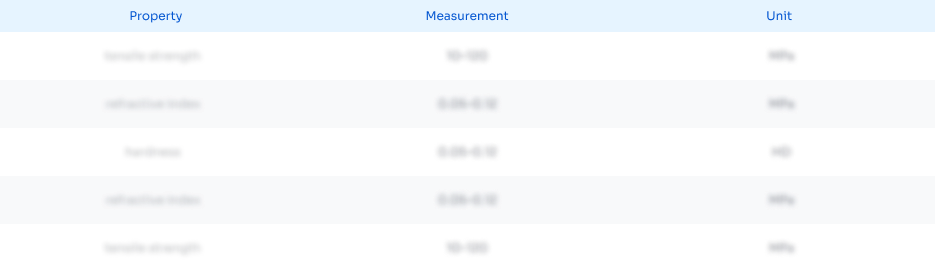
Abstract
Description
Claims
Application Information
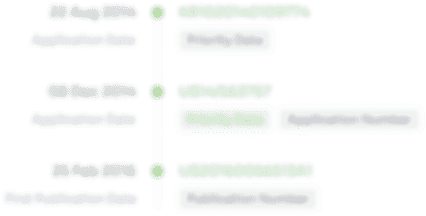
- R&D Engineer
- R&D Manager
- IP Professional
- Industry Leading Data Capabilities
- Powerful AI technology
- Patent DNA Extraction
Browse by: Latest US Patents, China's latest patents, Technical Efficacy Thesaurus, Application Domain, Technology Topic, Popular Technical Reports.
© 2024 PatSnap. All rights reserved.Legal|Privacy policy|Modern Slavery Act Transparency Statement|Sitemap|About US| Contact US: help@patsnap.com