A three-state energy control method based on automatic extended depth learning
A technology of deep learning and energy control, which is applied in the field of power generation scheduling and control in place of traditional multi-time scale scheduling and control, and can solve problems such as non-optimal scheduling, unit output with only one time scale, and reverse regulation. To achieve the effect of satisfying the constraints of the unit
- Summary
- Abstract
- Description
- Claims
- Application Information
AI Technical Summary
Problems solved by technology
Method used
Image
Examples
Embodiment Construction
[0030] A three-state energy control method based on automatic extended deep learning proposed by the present invention is described in detail in conjunction with the accompanying drawings as follows:
[0031] figure 1 is a schematic diagram of the scalable neural network of the method of the present invention. The number of neurons in the input layer, the 4 hidden layers, and the output layer are respectively {n i ,n 1 no i ,n 2 no i ,n 3 no 0 ,n 4 no 0 ,n 0}, where n i , n 0 Respectively, the number of neurons in the input layer and output layer; n 1 , n 2 , n 3 , n 4 are the scaling coefficients of each hidden layer, respectively. When the input layer expands to (n′ i +n i ), the output layer is extended to (n 0 '+n 0 ), the number of neurons in each layer becomes {n i '+n i ,n 1 (n i '+n i ),n 2 (n i '+n i ), n 3 (n 0 '+n 0 ),n 4 (n 0 '+n 0 ),n 0 '+n 0}.
[0032] The designed deep learning network is used to predict the frequency deviati...
PUM
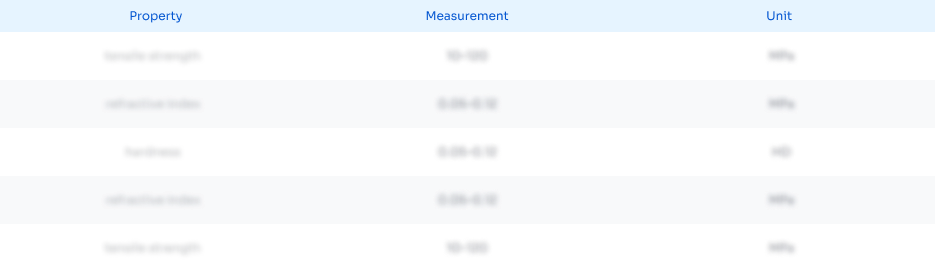
Abstract
Description
Claims
Application Information
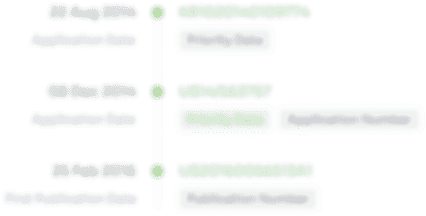
- R&D Engineer
- R&D Manager
- IP Professional
- Industry Leading Data Capabilities
- Powerful AI technology
- Patent DNA Extraction
Browse by: Latest US Patents, China's latest patents, Technical Efficacy Thesaurus, Application Domain, Technology Topic, Popular Technical Reports.
© 2024 PatSnap. All rights reserved.Legal|Privacy policy|Modern Slavery Act Transparency Statement|Sitemap|About US| Contact US: help@patsnap.com