Integrated kernel density estimator window parameter optimization method, device and terminal device
A technology of kernel density estimation and window parameters, which is applied in the field of window parameter optimization of integrated kernel density estimators, can solve the problems of inaccurate probability density function estimation and high time complexity
- Summary
- Abstract
- Description
- Claims
- Application Information
AI Technical Summary
Problems solved by technology
Method used
Image
Examples
Embodiment 1
[0075] Such as figure 1 As shown, the embodiment of the present invention provides an integrated kernel density estimator window parameter optimization method, including the following steps:
[0076] S101. Obtain random sample division data blocks based on the original data set.
[0077] In the above step S101, the original data set includes one-dimensional data and multi-dimensional data, and the data blocks divided by random samples include one or more data in the original data set.
[0078] Such as figure 2 As shown, the detailed implementation process of obtaining random sample division data blocks in the above step S101 may include:
[0079] S1011. Divide the original samples of the original data set to obtain original sample division data blocks.
[0080] In the above step S1011, a plurality of original samples are selected in the original data set, and the data is divided where there are original samples, so as to obtain original sample division data blocks of the o...
Embodiment 2
[0118] The embodiment of the present invention takes a one-dimensional original data set as an example to illustrate the implementation process of the method for optimizing the window parameters of the integrated kernel density estimator provided in the first embodiment.
[0119] Assuming an existing dataset in is the number of training samples in the data set D.
[0120] Assume that a random sample of the existing data set D is divided into data blocks in To meet the conditions:
[0121] 1,
[0122] 2. For any And k i ≠k j , established;
[0123] E[p k (x)]=p(x), where p k (x) and p(x) respectively divide the data block D for random samples k And the probability density function of the original data set D, E(X) is the expectation of the random variable X.
[0124] Then obtain the random sample partition data block of the original data set D Specifically:
[0125] First, through the original data set D in the sample The formula for direct division ope...
Embodiment 3
[0151] The embodiment of the present invention aims at the window parameter optimization method of the integrated kernel density estimator provided in the first embodiment, and uses test data to exemplify the beneficial effect in its practical application.
[0152] The embodiments of the present invention respectively adopt the random number data sets of normal distribution and exponential distribution to verify the convergence and test error of the integrated kernel density estimator.
[0153] Five RSP data blocks are randomly selected from the random number data sets of normal distribution and exponential distribution, each data block contains 100 samples as the training set and verification set, and 200 random samples are randomly generated as the test set. The number of iterations of the particle swarm optimization algorithm in the integrated kernel density estimator is 200, and the initial window width parameter of the integrated kernel density estimator is randomly select...
PUM
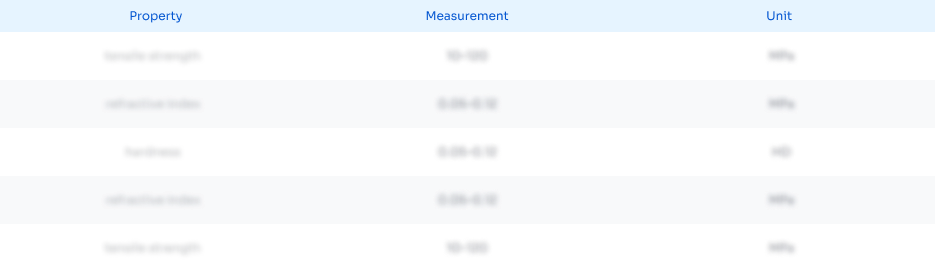
Abstract
Description
Claims
Application Information
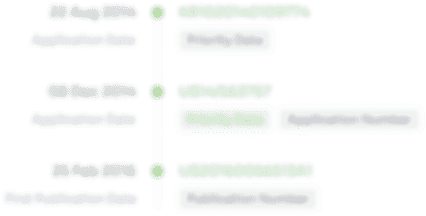
- R&D Engineer
- R&D Manager
- IP Professional
- Industry Leading Data Capabilities
- Powerful AI technology
- Patent DNA Extraction
Browse by: Latest US Patents, China's latest patents, Technical Efficacy Thesaurus, Application Domain, Technology Topic, Popular Technical Reports.
© 2024 PatSnap. All rights reserved.Legal|Privacy policy|Modern Slavery Act Transparency Statement|Sitemap|About US| Contact US: help@patsnap.com