Hybrid machine learning signal classification method based on PCA dimension reduction
A machine learning and signal classification technology, applied in the field of communication, can solve the problems of insufficient adaptability to unknown situations, low degree of automation, limited training samples, etc.
- Summary
- Abstract
- Description
- Claims
- Application Information
AI Technical Summary
Problems solved by technology
Method used
Image
Examples
Embodiment
[0137] MATLAB simulation is used to verify the classification and recognition performance of multi-class radar and communication signals, including two stages of training and testing.
[0138] In the training phase, the signal-to-noise ratio of the four signals is set to 20dB, and the symbol width is 10 -6 s, the number of sampling points is 5000, the sampling frequency is 100MHz, the carrier frequency is 20MHz, the chirp bandwidth is 10MHz, the time width is 50us, and the frequency of 2FSK is 10MHz and 20MHz respectively.
[0139] In the first level of training, the four signals are trained separately based on the instantaneous autocorrelation method. The instantaneous autocorrelation processing results of the four signals are as follows: Figure 4 , 5 , 6, and 7. After the instantaneous autocorrelation cycle is processed, the zero-crossing points of the four signals are cyclically extracted 50 times, and the results are as follows Figure 8 As shown, it can be seen that the number...
PUM
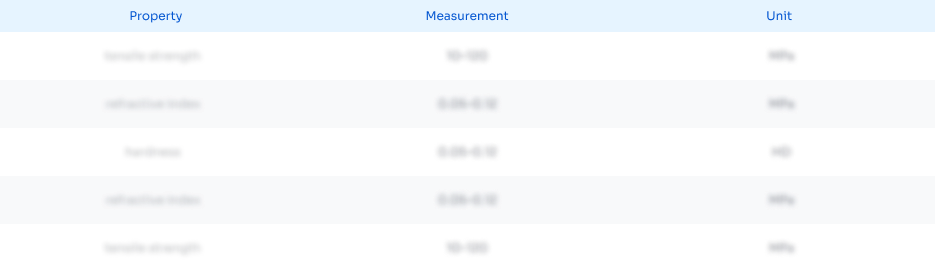
Abstract
Description
Claims
Application Information
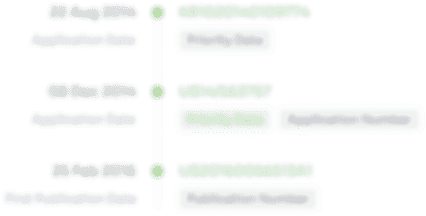
- R&D
- Intellectual Property
- Life Sciences
- Materials
- Tech Scout
- Unparalleled Data Quality
- Higher Quality Content
- 60% Fewer Hallucinations
Browse by: Latest US Patents, China's latest patents, Technical Efficacy Thesaurus, Application Domain, Technology Topic, Popular Technical Reports.
© 2025 PatSnap. All rights reserved.Legal|Privacy policy|Modern Slavery Act Transparency Statement|Sitemap|About US| Contact US: help@patsnap.com