Machine learning method used for electrolytic-cell fault early-warning and application thereof
A fault warning and machine learning technology, applied in the field of forecasting and machine learning clustering, can solve problems such as operator paralysis and equipment loss, achieve excellent model fitting effects, avoid judgment errors, and reduce paralysis
- Summary
- Abstract
- Description
- Claims
- Application Information
AI Technical Summary
Problems solved by technology
Method used
Image
Examples
Embodiment Construction
[0058] In order to make the technical problems, technical solutions and advantages to be solved by the present invention clearer, the specific implementation steps will be described in detail below.
[0059] Such as figure 1 As shown, the application of the GMM model in the early warning of electrolyzer failure is mainly realized through the following steps:
[0060] Step 1, data preparation, the data source used for modeling and analysis needs to be obtained through several steps:
[0061] Step 1.1, select the detection point, such as figure 2 As shown, according to experience, determine the relevant detection points that have an impact on the operation of the electrolytic cell. The selected detection points include the pressure difference between the positive and negative chambers of the electrolytic cell, the voltage difference between the front and rear ends of the electrolytic cell, the anode circulation flow, the cathode circulation flow, the supplementary brine flow, ...
PUM
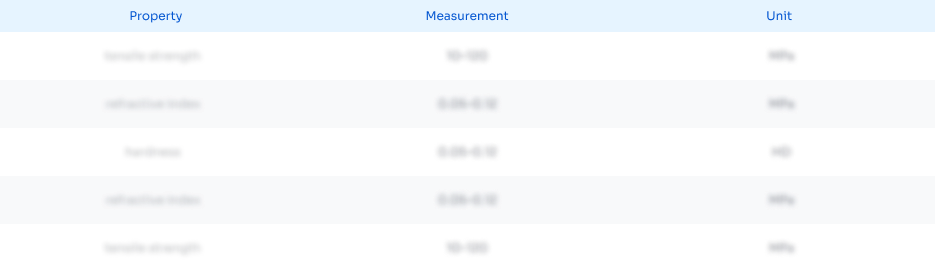
Abstract
Description
Claims
Application Information
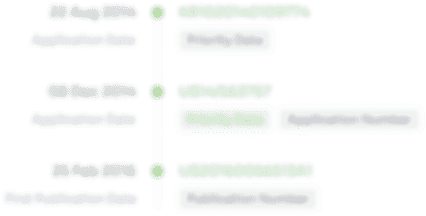
- R&D Engineer
- R&D Manager
- IP Professional
- Industry Leading Data Capabilities
- Powerful AI technology
- Patent DNA Extraction
Browse by: Latest US Patents, China's latest patents, Technical Efficacy Thesaurus, Application Domain, Technology Topic, Popular Technical Reports.
© 2024 PatSnap. All rights reserved.Legal|Privacy policy|Modern Slavery Act Transparency Statement|Sitemap|About US| Contact US: help@patsnap.com