A kind of truck danger reminding method
A truck and dangerous technology, applied in the field of truck safety early warning, can solve the problem of frequent accidents in blind areas of vision, and achieve the effects of fast recognition speed, fast early warning speed and high recognition efficiency
- Summary
- Abstract
- Description
- Claims
- Application Information
AI Technical Summary
Problems solved by technology
Method used
Image
Examples
Embodiment 1
[0075] Such as figure 1 Shown is a truck danger warning method according to an embodiment of the present invention, comprising the following steps:
[0076] S1. For a truck located in a mine area, each image acquisition device on the truck collects image information of a corresponding area.
[0077] S2. The image processing center connected to all image acquisition devices on the truck processes the image information in real time to determine whether there is a target to be identified within the preset range of the current truck;
[0078] Wherein, step S2 specifically includes:
[0079] S21. Perform preprocessing on the images collected by each image collection device, and obtain preprocessed images;
[0080] S22. Using the trained lightweight SSD model to process the preprocessed image in real time;
[0081] Wherein, the lightweight SSD model after training is obtained by training the lightweight SSD model with training images related to the current truck in advance.
[0...
Embodiment 2
[0098] When running for the first time the program carrying a truck danger reminder method of the present invention, it is necessary to train the lightweight SSD model, and the training of the lightweight SSD model specifically includes the following steps:
[0099] L01: Input the preprocessed training image into the lightweight SSD model to obtain the first feature map;
[0100] It should be noted that, in order to perform detection on multiple scales, the first feature maps of the new module, the seventh module and the eighth module are extracted here. Each first feature map contains a certain number of default bounding boxes, i.e., default boxes, which have a certain scale and aspect ratio, and two different types of filters (i.e., position and score) are applied to each first feature Graph to predict the location offset and target score of the default box.
[0101] L02: Calculate the first default frame of each first feature map;
[0102] First, the first feature map is ...
Embodiment 3
[0133] The trained lightweight SSD model structure is:
[0134] The input of the lightweight SSD model is a 300×300×3 image, the number of channels of the image is 3, and it is an RGB (three primary colors of image color: red, green, blue) image. The first module: first there are two convolution layers, the size of the convolution kernel is 3×3, the number of convolution kernels is 64, the step size is 1, and the padding method is 'SAME' (zero padding makes the input and output images the same size ), the activation function is a linear rectification function (Rectified Linear Unit, ReLU), and then the maximum pooling layer, the sampling kernel size is 2×2, the step size is 2, and the output is 64 feature maps of 150×150;
[0135] The second module: first there are two convolutional layers, the convolution kernel size is 3×3, the number of convolution kernels is 128, and then the maximum pooling layer, the sampling kernel size is 2×2, and the output is 128 75×75 feature map; ...
PUM
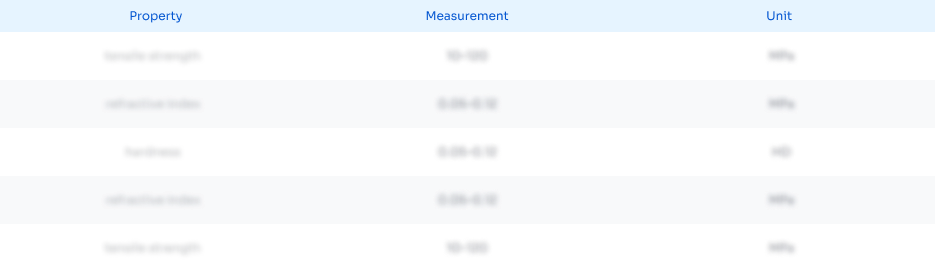
Abstract
Description
Claims
Application Information
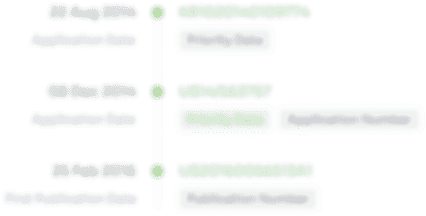
- R&D Engineer
- R&D Manager
- IP Professional
- Industry Leading Data Capabilities
- Powerful AI technology
- Patent DNA Extraction
Browse by: Latest US Patents, China's latest patents, Technical Efficacy Thesaurus, Application Domain, Technology Topic, Popular Technical Reports.
© 2024 PatSnap. All rights reserved.Legal|Privacy policy|Modern Slavery Act Transparency Statement|Sitemap|About US| Contact US: help@patsnap.com