Variational Bayesian self-adaptive filtering method
A variational Bayesian and adaptive filtering technology, applied in the field of filter subsystems, can solve the problems of unknown process noise, divergence, and large estimation error, etc., and achieve the effect of improving tracking accuracy
- Summary
- Abstract
- Description
- Claims
- Application Information
AI Technical Summary
Problems solved by technology
Method used
Image
Examples
Embodiment Construction
[0038] Now in conjunction with embodiment, accompanying drawing, the present invention will be further described:
[0039] figure 1 It is a flow chart of a variational Bayesian adaptive filtering method of the present invention, and the specific implementation details of each part are as follows:
[0040] 1. Adaptive filtering problem description
[0041] The state equation and measurement of the target in linear space are:
[0042] x k =F k x k-1 +w k
[0043] the y k =H k x k +v k
[0044] Among them, the state transition matrix F k and measure H k is given, the process noise w k Gaussian white noise with zero mean and covariance Q k Time-varying and unknown, the measurement noise v k Gaussian white noise with zero mean, its noise covariance R k >0 is known. initial state x 0 is the known mean x 0|0 and covariance P 0|0 Gaussian distribution.
[0045] According to the Kalman filter, the prior distribution of the target state is
[0046]
[0047] in,...
PUM
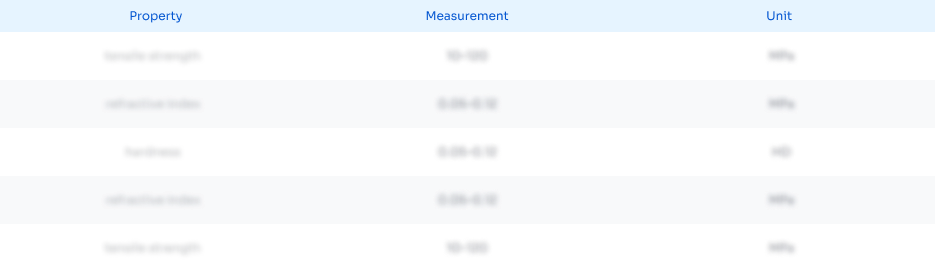
Abstract
Description
Claims
Application Information
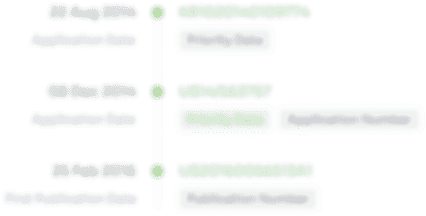
- R&D Engineer
- R&D Manager
- IP Professional
- Industry Leading Data Capabilities
- Powerful AI technology
- Patent DNA Extraction
Browse by: Latest US Patents, China's latest patents, Technical Efficacy Thesaurus, Application Domain, Technology Topic, Popular Technical Reports.
© 2024 PatSnap. All rights reserved.Legal|Privacy policy|Modern Slavery Act Transparency Statement|Sitemap|About US| Contact US: help@patsnap.com