Deep learning method for predicting urban traffic jam based on GPS
A technology of deep learning and urban traffic, applied in the direction of neural learning methods, traffic flow detection, traffic control system of road vehicles, etc., can solve the problems of limiting the practicability of research results, ignoring the complex traffic road topology, etc., to achieve practicality strong, good effect
- Summary
- Abstract
- Description
- Claims
- Application Information
AI Technical Summary
Benefits of technology
Problems solved by technology
Method used
Image
Examples
Embodiment Construction
[0048] The preferred embodiments of the GPS-based deep learning method for predicting urban traffic congestion of the present invention will be described in detail below with reference to the accompanying drawings.
[0049] Figure 1 to Figure 7 Show the specific implementation of the GPS-based urban traffic congestion prediction deep learning method of the present invention:
[0050] like figure 1 As shown, the GPS-based deep learning method for predicting urban traffic congestion includes the following steps:
[0051] (1) Store the original GPS data generated by the driver in the process of using the mobile phone terminal navigation software, wherein the original GPS data includes the field information of time, latitude and longitude, user id, speed, and direction angle;
[0052](2) Traffic network characterization. Traffic network characterization is divided into three specific steps. First, the urban transportation network considering the time dimension is transformed i...
PUM
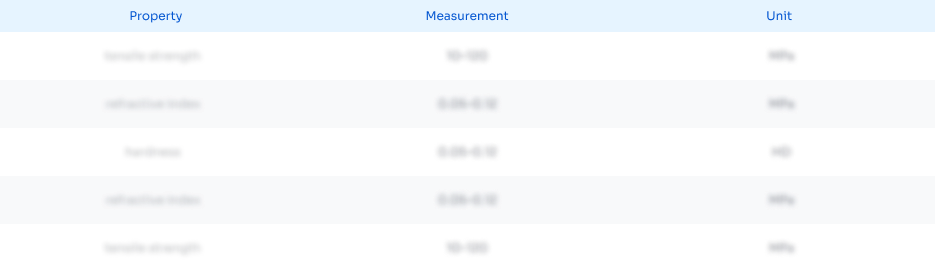
Abstract
Description
Claims
Application Information
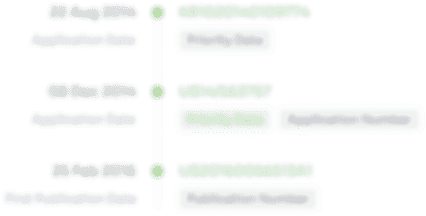
- R&D Engineer
- R&D Manager
- IP Professional
- Industry Leading Data Capabilities
- Powerful AI technology
- Patent DNA Extraction
Browse by: Latest US Patents, China's latest patents, Technical Efficacy Thesaurus, Application Domain, Technology Topic, Popular Technical Reports.
© 2024 PatSnap. All rights reserved.Legal|Privacy policy|Modern Slavery Act Transparency Statement|Sitemap|About US| Contact US: help@patsnap.com