Hyper-parameter automatic optimization method and system of non-supervised machine learning
A machine learning and automatic optimization technology, applied in the computer field, can solve the problem of low optimization efficiency of hyperparameter optimization methods, and achieve the effect of reducing the complexity of use and high optimization efficiency.
- Summary
- Abstract
- Description
- Claims
- Application Information
AI Technical Summary
Problems solved by technology
Method used
Image
Examples
Embodiment 1
[0036] figure 1 It is a schematic diagram of an automatic hyperparameter optimization method for unsupervised machine learning according to an embodiment of the present invention, such as figure 1 As shown, the embodiment of the present invention provides a hyperparameter automatic optimization method for unsupervised machine learning, including:
[0037] Step S10, determining the algorithm performance evaluation model of the unsupervised machine learning algorithm, the hyperparameters of the unsupervised machine learning algorithm, the search space of the hyperparameters, and the evaluation criteria for hyperparameter optimization according to the unsupervised machine learning algorithm ;
[0038] Step S20, determining the optimal value of the hyperparameter according to the algorithm performance evaluation model, the search space and the evaluation criterion.
[0039] Further, according to the unsupervised machine learning algorithm, the algorithm performance evaluation mo...
Embodiment 2
[0097] figure 2 It is a schematic diagram of an automatic hyperparameter optimization system for unsupervised machine learning according to an embodiment of the present invention, such as figure 2 As shown, the embodiment of the present invention provides a hyperparameter automatic optimization system of unsupervised machine learning, which is used to complete the method in the above embodiment, and specifically includes a query module 10 and an optimization module 20, wherein,
[0098] The query module 10 is used to determine the algorithm performance evaluation model of the unsupervised machine learning algorithm, the hyperparameters of the unsupervised machine learning algorithm, the search space of the hyperparameters and the optimization parameters of the hyperparameters according to the unsupervised machine learning algorithm. evaluation criteria;
[0099] The optimization module 20 is used to determine the optimal value of the hyperparameter according to the algorith...
Embodiment 3
[0102] image 3 A schematic structural diagram of an electronic device for automatically optimizing hyperparameters of unsupervised machine learning provided by an embodiment of the present invention, such as image 3 As shown, the device includes: a processor 801, a memory 802 and a bus 803;
[0103] Wherein, the processor 801 and the memory 802 complete mutual communication through the bus 803;
[0104] The processor 801 is used to call the program instructions in the memory 802 to execute the methods provided by the above method embodiments, including, for example:
[0105] Determine the algorithm performance evaluation model of the unsupervised machine learning algorithm, the hyperparameters of the unsupervised machine learning algorithm, the search space of the hyperparameters, and the evaluation criteria for hyperparameter optimization according to the unsupervised machine learning algorithm;
[0106] The optimal value of the hyperparameter is determined according to t...
PUM
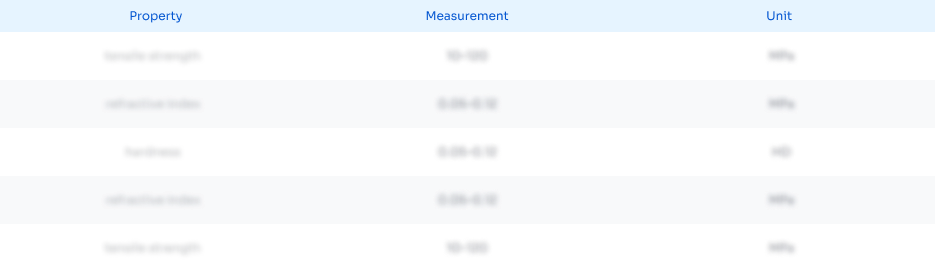
Abstract
Description
Claims
Application Information
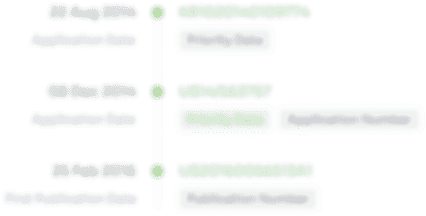
- R&D Engineer
- R&D Manager
- IP Professional
- Industry Leading Data Capabilities
- Powerful AI technology
- Patent DNA Extraction
Browse by: Latest US Patents, China's latest patents, Technical Efficacy Thesaurus, Application Domain, Technology Topic, Popular Technical Reports.
© 2024 PatSnap. All rights reserved.Legal|Privacy policy|Modern Slavery Act Transparency Statement|Sitemap|About US| Contact US: help@patsnap.com