Full-reference image quality evaluation method based on image distortion types
A technology for image quality evaluation and image distortion, applied in image communication, television, electrical components, etc., it can solve the problems that the algorithm performance is not excellent, the subjective evaluation of the evaluation model cannot be highly consistent, and achieve the effect of excellent performance
- Summary
- Abstract
- Description
- Claims
- Application Information
AI Technical Summary
Problems solved by technology
Method used
Image
Examples
Embodiment Construction
[0027] Embodiments of the present invention will be further described in detail below in conjunction with the accompanying drawings.
[0028] The overall idea of the present invention is to integrate four different theoretical full-reference image quality evaluation methods to generate a new hybrid image quality model, and then use the convolutional neural network model to judge the distortion type of the distorted image. Next, according to the distortion type, select The corresponding image quality evaluation expression is used to obtain the objective quality evaluation score. The specific system method flow is mainly realized through the following main steps:
[0029] The first step is to select images of four types of distortion (Gaussian white noise, Gaussian blur, JPEG compression, and JPEG2000 compression) in the image library TID2008 as experimental samples, and perform local normalization processing on the sample distorted images. The specific processing process is a...
PUM
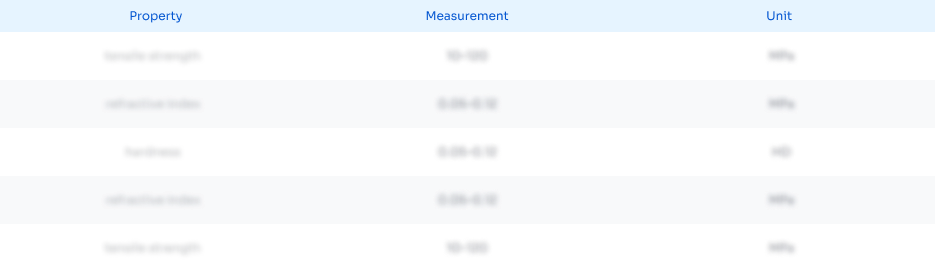
Abstract
Description
Claims
Application Information
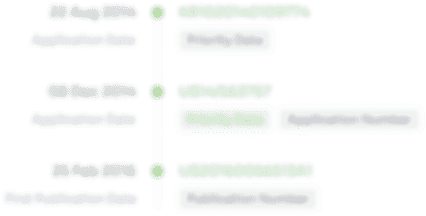
- R&D Engineer
- R&D Manager
- IP Professional
- Industry Leading Data Capabilities
- Powerful AI technology
- Patent DNA Extraction
Browse by: Latest US Patents, China's latest patents, Technical Efficacy Thesaurus, Application Domain, Technology Topic, Popular Technical Reports.
© 2024 PatSnap. All rights reserved.Legal|Privacy policy|Modern Slavery Act Transparency Statement|Sitemap|About US| Contact US: help@patsnap.com