Dynamic texture recognition method based on multi-task learning
A multi-task learning, dynamic texture technology, applied in character and pattern recognition, instruments, computer parts and other directions, can solve problems such as complex computing, and achieve the effect of improving learning efficiency
- Summary
- Abstract
- Description
- Claims
- Application Information
AI Technical Summary
Problems solved by technology
Method used
Image
Examples
Embodiment Construction
[0056] The specific implementation manners of the present invention will be further described in detail below in conjunction with the accompanying drawings and embodiments. The following examples are used to illustrate the present invention, but are not intended to limit the scope of the present invention.
[0057] Previous recognition methods, such as sparse representation methods, learn each histogram feature, which can be regarded as a single-task learning process. Simultaneously solving sparse representations for multiple training features can be seen as a multi-task learning process. Based on this idea, the present invention proposes to use the dirty model to solve, such as Figure 5 shown. Decompose the total model W into group sparse model parts l 1,∝ and element sparse part l 1,1 . The group sparse model part can obtain the common features between multiple tasks, while the element sparse model can obtain the individual parts of each feature. Therefore, the mixtur...
PUM
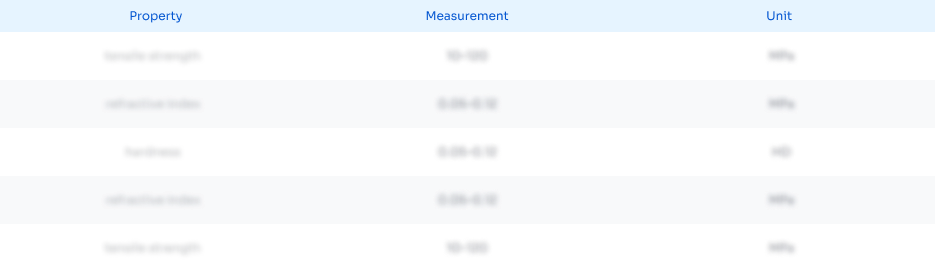
Abstract
Description
Claims
Application Information
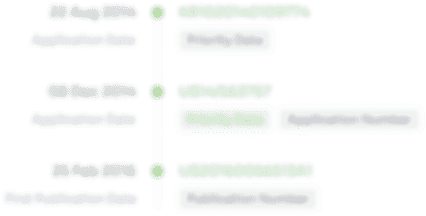
- R&D Engineer
- R&D Manager
- IP Professional
- Industry Leading Data Capabilities
- Powerful AI technology
- Patent DNA Extraction
Browse by: Latest US Patents, China's latest patents, Technical Efficacy Thesaurus, Application Domain, Technology Topic, Popular Technical Reports.
© 2024 PatSnap. All rights reserved.Legal|Privacy policy|Modern Slavery Act Transparency Statement|Sitemap|About US| Contact US: help@patsnap.com