Recommendation method for graph construction and L1 regular matrix decomposition combination learning
A matrix decomposition and recommendation method technology, applied in the field of recommendation learning, can solve the problems affecting the performance of the recommendation algorithm, difficulty in parameter selection, and not necessarily effective, and achieve the effect of solving the problem of data sparsity, accurate hidden semantic features, and fast convergence
- Summary
- Abstract
- Description
- Claims
- Application Information
AI Technical Summary
Problems solved by technology
Method used
Image
Examples
Embodiment Construction
[0039] Embodiments of the invention are described in detail below, examples of which are illustrated in the accompanying drawings. The embodiments described below by referring to the figures are exemplary only for explaining the present invention and should not be construed as limiting the present invention.
[0040] Aiming at the recommendation problem based on scoring data, the present invention focuses on the local similarity between items and the joint learning model construction of matrix decomposition, and uses graphs to describe the similarity relationship between items, especially assuming that the latent semantic features of users and items obey the Lapp Lass distribution, in order to better meet the sparse nature, construct a collaborative filtering recommendation method for joint learning of graph edge weights and hidden semantic features.
[0041] like figure 1 As shown, the present invention discloses a recommendation method for joint learning of graph constructi...
PUM
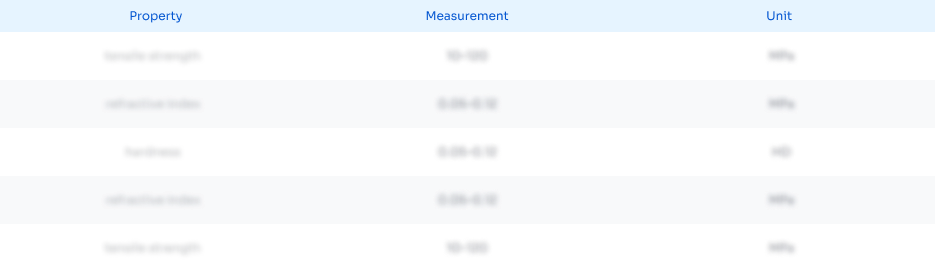
Abstract
Description
Claims
Application Information
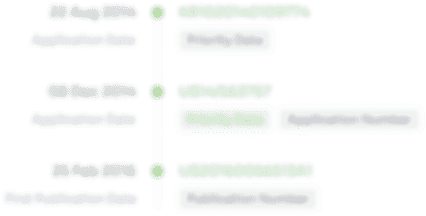
- R&D Engineer
- R&D Manager
- IP Professional
- Industry Leading Data Capabilities
- Powerful AI technology
- Patent DNA Extraction
Browse by: Latest US Patents, China's latest patents, Technical Efficacy Thesaurus, Application Domain, Technology Topic, Popular Technical Reports.
© 2024 PatSnap. All rights reserved.Legal|Privacy policy|Modern Slavery Act Transparency Statement|Sitemap|About US| Contact US: help@patsnap.com