A Robust k-means Clustering Method for Power User Segmentation
A technology of power users and clustering methods, applied in the field of robust k-means clustering, which can solve the limitation of labor cost and artificial understanding depth, cannot realize user classification, cannot realize accurate, fast and detailed classification of users, etc. problems, to achieve the effect of reliable clustering results, insensitive selection, and high accuracy
- Summary
- Abstract
- Description
- Claims
- Application Information
AI Technical Summary
Problems solved by technology
Method used
Image
Examples
Embodiment 1
[0025] A kind of robust k-means clustering method oriented to electric power user subdivision of the present invention, comprises the following steps, step 1: extract any group of data sets of electric power company, carry out data standardization processing, described data set is made up of a plurality of clusters; Step 2: Extract the standardized data set, and calculate the dissimilarity between samples in the data set; Step 3: Extract the dissimilarity between samples in the data set in step 2, use the improved MaxMin initialization method to select the initial cluster center, and determine The number and type of cluster centers; step 4: according to the number and type of cluster centers in step 3, automatically split or merge clusters.
[0026] The normalization processing methods in step 1 include maximum and minimum normalization, z-score normalization and decimal scaling normalization.
[0027]In the step 2, the dissimilarity between samples in the data set is calculat...
Embodiment 2
[0052] A robust k-means clustering method for electric power user subdivision, including the following steps, step 1: extract any set of data sets of electric power companies, and perform data standardization processing, the data set is composed of multiple clusters; step 2 : Extract the data set after standardized processing, and calculate the dissimilarity between samples in the data set; Step 3: Extract the dissimilarity between samples in the data set in step 2, use the improved MaxMin initialization method to select the initial cluster center, and determine the cluster The number and type of centers; step 4: according to the number and type of cluster centers in step 3, automatically split or merge clusters.
[0053] The normalization processing methods in step 1 include maximum and minimum normalization, z-score normalization and decimal scaling normalization.
[0054] In the step 2, the dissimilarity between samples in the data set is calculated, and when the data sampl...
PUM
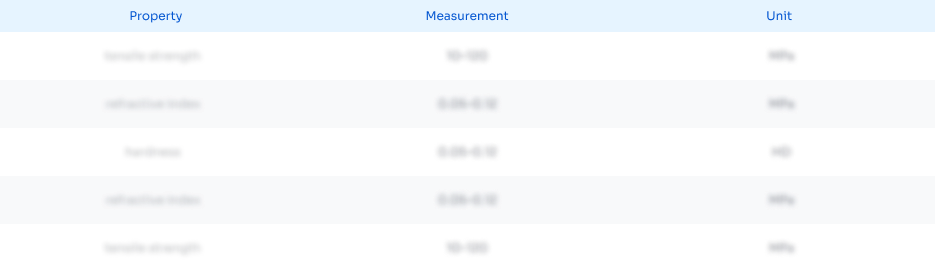
Abstract
Description
Claims
Application Information
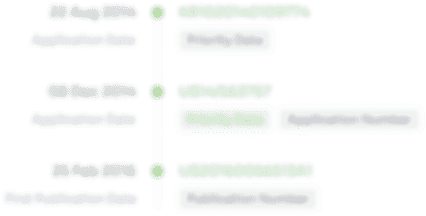
- R&D Engineer
- R&D Manager
- IP Professional
- Industry Leading Data Capabilities
- Powerful AI technology
- Patent DNA Extraction
Browse by: Latest US Patents, China's latest patents, Technical Efficacy Thesaurus, Application Domain, Technology Topic, Popular Technical Reports.
© 2024 PatSnap. All rights reserved.Legal|Privacy policy|Modern Slavery Act Transparency Statement|Sitemap|About US| Contact US: help@patsnap.com