A target recognition method based on smooth multi-instance learning
A multi-instance learning and target recognition technology, applied in character and pattern recognition, image analysis, image enhancement, etc., can solve the problems of failing to obtain a good recognition effect, heavy relocation, time-consuming and labor-intensive, etc., and achieve short recognition time. , good effect, high efficiency effect
- Summary
- Abstract
- Description
- Claims
- Application Information
AI Technical Summary
Problems solved by technology
Method used
Image
Examples
Embodiment Construction
[0051] The present invention will be described in detail below in conjunction with the accompanying drawings and specific embodiments. This embodiment is carried out on the premise of the technical solution of the present invention, and detailed implementation and specific operation process are given, but the protection scope of the present invention is not limited to the following embodiments.
[0052] The flow chart of the target recognition method based on smooth multi-instance learning is as follows: figure 1 shown. The method includes a sample training step and a target recognition step, and the sample training step is specifically:
[0053] A1) Extract the example features of the training picture:
[0054] A11) take each training picture as a training bag, produce the example corresponding to each training bag by Edgebox;
[0055] A12) Perform feature extraction on all examples generated in step A11), and obtain the feature value x of each example ij ;
[0056] A2) ...
PUM
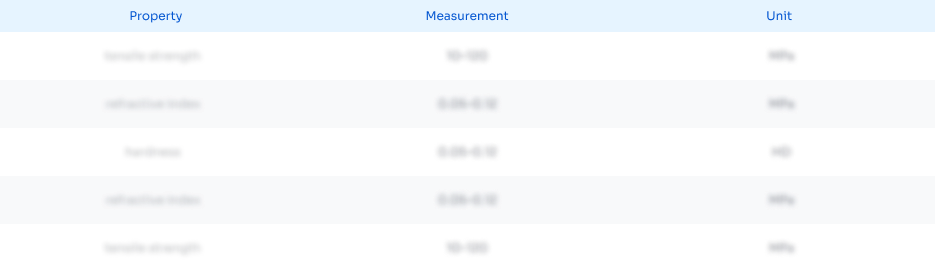
Abstract
Description
Claims
Application Information
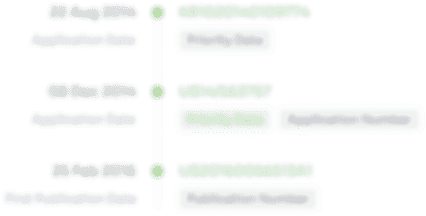
- R&D Engineer
- R&D Manager
- IP Professional
- Industry Leading Data Capabilities
- Powerful AI technology
- Patent DNA Extraction
Browse by: Latest US Patents, China's latest patents, Technical Efficacy Thesaurus, Application Domain, Technology Topic, Popular Technical Reports.
© 2024 PatSnap. All rights reserved.Legal|Privacy policy|Modern Slavery Act Transparency Statement|Sitemap|About US| Contact US: help@patsnap.com