Zero sample classification method based on multi-mode dictionary learning
A technology of dictionary learning and classification methods, applied in the field of zero-sample classification, can solve problems such as the inability to fully represent the semantics of categories, achieve simple and efficient practicality, and improve training efficiency
- Summary
- Abstract
- Description
- Claims
- Application Information
AI Technical Summary
Benefits of technology
Problems solved by technology
Method used
Image
Examples
Embodiment Construction
[0036] A zero-shot classification method based on multimodal dictionary learning of the present invention will be described in detail below with reference to the embodiments and drawings.
[0037] A zero-sample classification method based on multi-modal dictionary learning of the present invention is a basic framework for using dictionary learning for zero-sample classification to solve the problem of poor expression ability of category semantic features in zero-sample classification. The idea is to use the training samples to learn a shared dictionary matrix to map the samples from the visual space to the implicit space formed by the dictionary atoms. Each dictionary atom represents an implicit attribute feature, and the embedded features of the sample in the hidden space It is more Lupine-like for changes in samples within a class. And use the representation of the training samples in the latent space, the category semantic features corresponding to the samples and the corre...
PUM
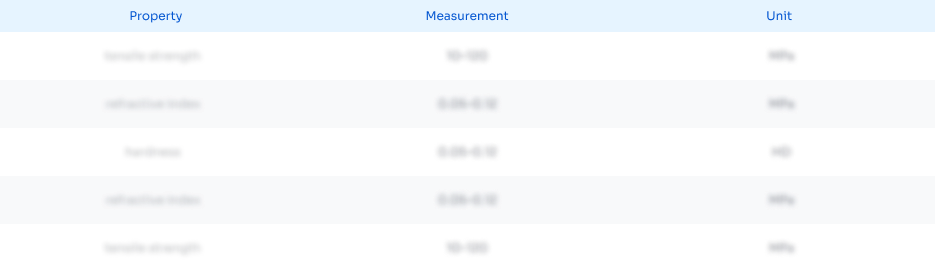
Abstract
Description
Claims
Application Information
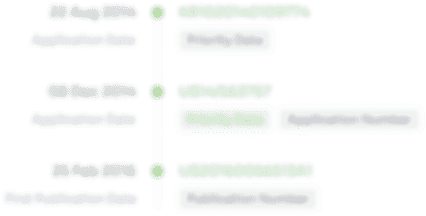
- R&D Engineer
- R&D Manager
- IP Professional
- Industry Leading Data Capabilities
- Powerful AI technology
- Patent DNA Extraction
Browse by: Latest US Patents, China's latest patents, Technical Efficacy Thesaurus, Application Domain, Technology Topic, Popular Technical Reports.
© 2024 PatSnap. All rights reserved.Legal|Privacy policy|Modern Slavery Act Transparency Statement|Sitemap|About US| Contact US: help@patsnap.com